According to Gartner, only 16% of decision-makers feel that financial data can be easily leveraged for decision-making. Automation and AI make data more actionable, helping today’s savvy financial companies extract and apply data more effectively. But getting your model out of development and into production so you can reap those benefits can be a challenge.
In our latest webinar, Improve Your Finance AI Strategy with Humans in the Loop, CloudFactory CTO, Pieter Nel, shares an overview of how AI and automation are impacting the financial industry and how to overcome impediments in your machine learning and AI projects. During the webinar, he goes into depth on how to:
- Leverage key drivers in fintech modernization
- Include humans in the loop (HITL) in your AI and automation strategy
- Determine the right technology and tooling for your unique use case
- Build from real-life finance AI and automation success stories
- Choose the right partner to ensure vetted and trained data analysts work on your project
If you don't have time to watch the entire webinar, this blog post summarizes the current bottlenecks in automation and AI for finance as well as the three most important takeaways.
Top bottlenecks in automation and AI for finance
Financial companies are actively pursuing two primary evolution paths with respect to leveraging data to improve operations and customer service:
- Automation: Leveraging structured and unstructured data that exist in different forms and locations. Humans and machines must gather, process, transcribe, and enrich it to add value.
- AI: Utilizing unstructured computer vision (CV) data. Humans and machines need to label and feed it into ML models, QA it, and continually refresh it as models evolve.
For most financial companies, the most common use cases for leveraging automation and AI are document processing, fraud detection, risk assessment, and claims processing automation.
With both automation and AI projects, humans and machines are integral to transforming the data into something you can extract value from. And that human component is one of the biggest roadblocks for most financial firms to truly capitalize on their automation and AI strategies.
For data-intensive projects in the finance space, ensuring the confidentiality of client personal information (PI) makes data security and integrity of critical importance when considering their workforce.
In fact, finding those skilled people is the top bottleneck to AI adoption, according to O’Reilly’s AI Adoption in the Enterprise survey, followed closely by lack of data or data quality issues.
The best way to address those bottlenecks? The right data partner.
3 ways to improve your finance AI strategy
Ready to take hold of your financial AI strategy and overcome impediments? Here are the top three takeaways Pieter shared in the webinar.
1. Start now: Putting off your AI adoption will impact your competitiveness.
According to McKinsey’s report, The State of AI in 2022, AI adoption has doubled in the last five years but has plateaued around 50-60%. Across all industries, including finance, robotic process automation, and CV are the leading AI capabilities. When looking at financial services specifically, O’Reilly’s survey states financial services is an industry most ready for AI transformation, only behind computers, electronics, and technology.
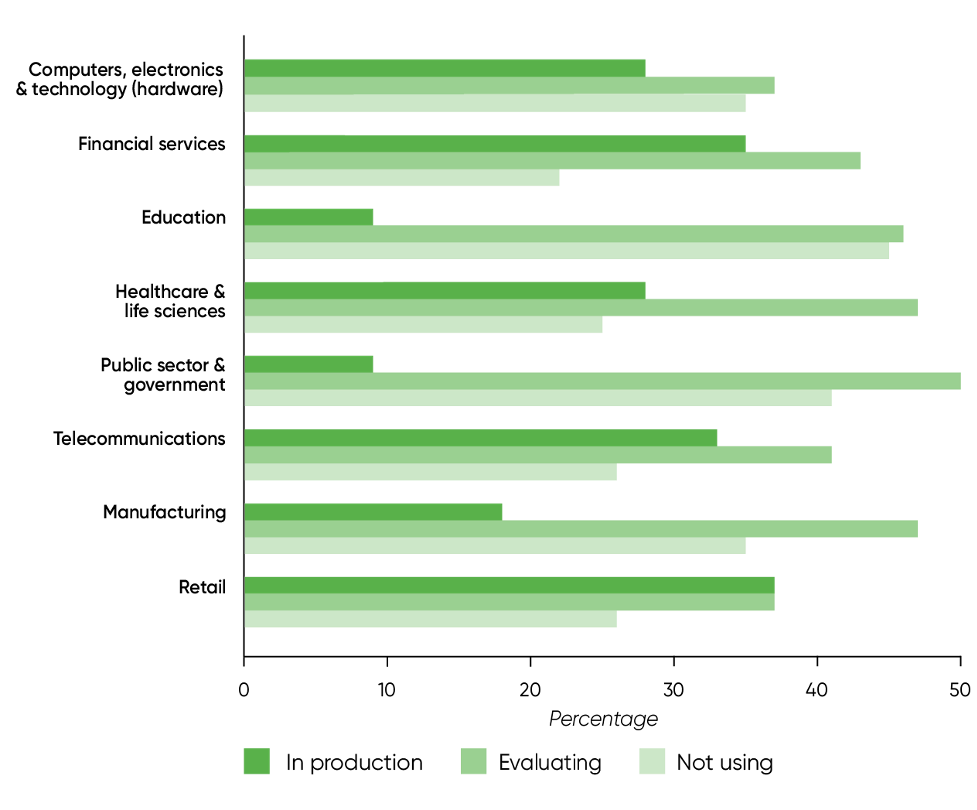
If you haven’t embedded AI capabilities into your products or business processes in at least one function or business unit, you run a high risk of being left behind and becoming so inefficient in your operations that you may not remain competitive. The good news: with the plateau, you still have time to catch up. And for those that have started, you have an opportunity to forge ahead while the rest of the market gets up to speed.
2. Understand the value of humans: The right people, processes, and technology are available to overcome your AI adoption friction.
When it comes to accelerating your machine learning (ML) and AI projects, HITL are essential. Data drives every stage of the model development process, and humans drive the data cleaning, annotation, quality control, and exception handling.
-
Design and build:
Enormous amounts of quality data are required to train models. For example, if you’re looking to train a model that will automate the processing of one type of document, you easily need 50K-100K examples that need to be labeled and annotated by humans in extreme detail. That can be a huge barrier. -
Deploy and operationalize:
Unique annotation requirements exist even within similar use cases. It’s essential to train the model on what is happening in real life and that means accounting for exceptions you didn’t anticipate. -
Refine and optimize:
Once in production, you still have to frequently update to account for model drift that happens as new variants of data emerge over time.
Every aspect of model development and deployment is labor intensive, requiring HITL.
3. Leverage experience: Partner with a company that has proven experience to help in your strategy.
If you want to scale AI projects at your financial company, you’ll need a data partner. Look for an experienced company like CloudFactory that embraces the shift from model-centric to data-centric ML. Following a data-centric approach means working on the model and data in tandem, allowing for rapid feedback and getting to market much faster at a lower cost.
This means you no longer have to sacrifice quality for efficiency. By employing human intervention on low-confidence data, you are quickly able to ramp up your quality. CloudFactory helps financial companies like Expensify, Ibotta, and Carpe Data integrate HITL and technology to offer a true end-to-end solution.
Data is critical to finance AI projects
When AI and automation projects fail, data is almost always the culprit. In fact, 96% of failures are due to data quality, data labeling, and unreliable models. Overcoming that barrier is key to getting your project into production and delivering value for your financial company. Want to learn more about building a successful finance AI strategy and taking control of your data? Watch the full webinar.