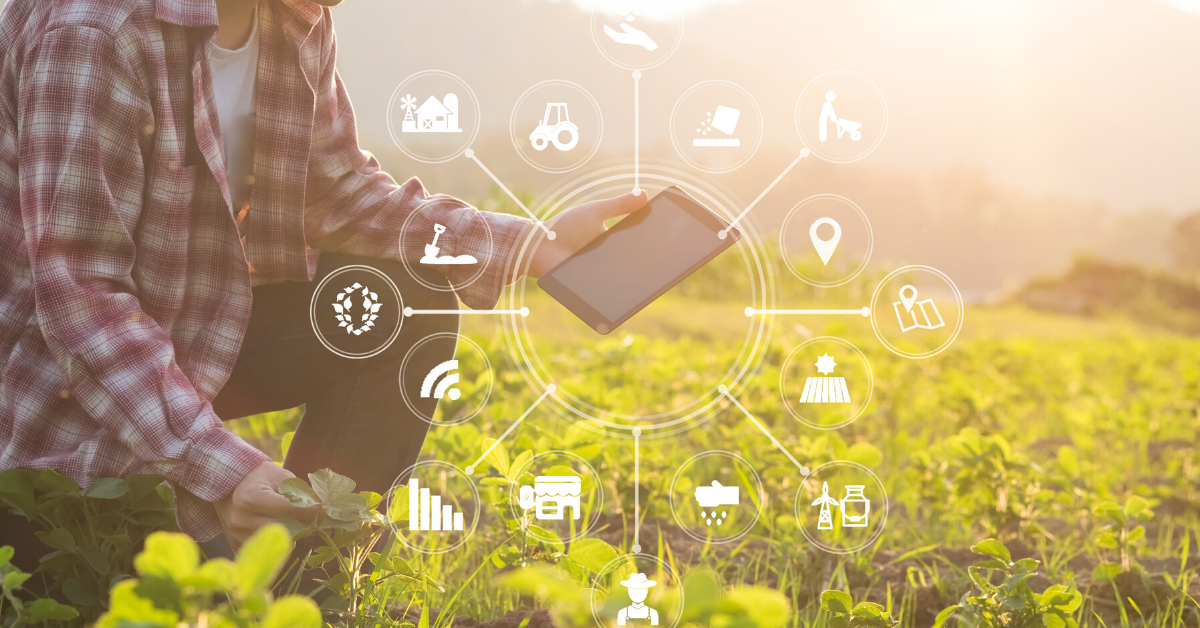
Technology holds great promise in several centuries-old industries, including agriculture. Automation and AI can help solve many of the challenges and inefficiencies in the global production and distribution of food by increasing crop yields, improving sustainability, reducing agrochemical input costs, and much more.
Agriculture technology companies are faced with a host of challenges due to the nature of their industry, things like seasonality, regional differences, and technology adoption. Added to those challenges is the highly variable, technical nature of the labeling work, which is often completed by data scientists in collaboration with staff agronomists. The level of agricultural expertise needed to complete the labeling work can be a huge barrier to agtech product development.
However, some agriculture technology companies are discovering that a dedicated, managed team can be trained to perform quality labeling work for these highly-technical tasks. These companies are able to free up valuable data science and agronomist resources so they can scale their business.
We spoke with one of our clients, Hummingbird Technologies, a crop analytics company about the importance of accurate labeling, using an external workforce, and the future of AI in agriculture.
How critical is accurate labeling to AI and machine learning in agriculture?
In our specific case, our AI models are providing information directly to decision-makers in agriculture. Therefore, it is important that the information is reliable since you don’t get a second chance. If a farmer trusts you, they need to be sure that crops will still perform the same after they take the suggested action from our products, as a loss of yield could result in grave consequences for their financial health. This could also lead to a much more dramatic impact on a regional scale. For this reason, the farming community is understandably cautious when investigating new technologies.
The problem with labeling is that agronomists are far too in demand in the fields to be able to assist us. The second best solution is that we consult them and draw a list of requirements together before we teach annotators. The annotators are then advised to flag every doubt during the labeling process so a data scientist or agronomist can help make a decision. While we cannot totally exclude human error during a labeling process, AI techniques allow us to generalize main rules from the data, meaning all one-off mistakes will be ignored. Regardless of this tolerance for error, it is critical to have the best annotations possible so that repeated errors do not occur and impact the model.
How do you think AI is going to evolve to help agriculture?
I think that the farming community will soon become more comfortable and knowledgeable about the potential for AI and AI-based, remote sensing products. The sort of Holy Grail that we always get asked about is pre-symptomatic disease detection. That is incredibly hard, if not impossible, to do at the moment. But we definitely see this happening with more sophisticated technology and greater improvements and accuracy. You can go from being right 85-90% of the time, but our customers will want 98%, 99% of the time. And that will definitely, definitely happen.
We're already setting the state-of-the-art in several products and we are very confident that within the coming years, the products that Hummingbird and CloudFactory develop will be seen as the industry standard. And then, in my opinion, if you're not using them you'll be seen as behind the times. It’s going to come to the point where, if you're using the industry practices that remain as standard in early 2020, then by 2023 you won’t survive.
Interview conducted with Hummingbird Technologies CMO, Alexander Jevons, and Senior Data Scientist, Francois Lemarchand.
Data Labeling Computer Vision AI & Machine Learning Agriculture