At CloudFactory we have a pretty intimate seat at the table with well over 100 active tech teams applying AI to a myriad of different use cases and industries. Our clients come in all sizes and from all industries, from small startups to those listed on the Fortune 500, and they work on solutions from cashierless checkout to self-driving cars.
With all the AI hype, it sometimes feels like a gold rush, which would make CloudFactory’s workforce solutions the picks and shovels. From those trenches, here are a few of our predictions for what 2021 will hold for AI and machine learning:
1. Organizations already using AI will break away from the pack.
We see no signs of an AI winter. Both AI adoption and business value will increase over the next 12 months. This will happen even wider across industries and deeper within enterprises.
But the growth and increase won’t be evenly distributed. There is a massive variance in AI program maturity and the levels of investment being made. It is easier than ever to prototype a model, and more people are doing just that every day, with emerging tools and frameworks accelerating the ubiquity of machine learning. There is no question the “pack” of people and companies developing AI is quickly getting larger.
Gartner depicts businesses’ adoption and use of AI in its AI Maturity Model. Companies move from early interest in AI to experimentation to AI in production to transformation, until AI is part of that business’ DNA.
The organizations that have cut through the hype and have AI models in production are the ones that will break away from the pack in 2021. They have escaped proof-of-concept purgatory, and even in a pandemic, they are investing more in AI and pushing ahead of the pack. We see this with many of our clients, who have found their product-market fit and pressing on to label more data and innovate even faster.
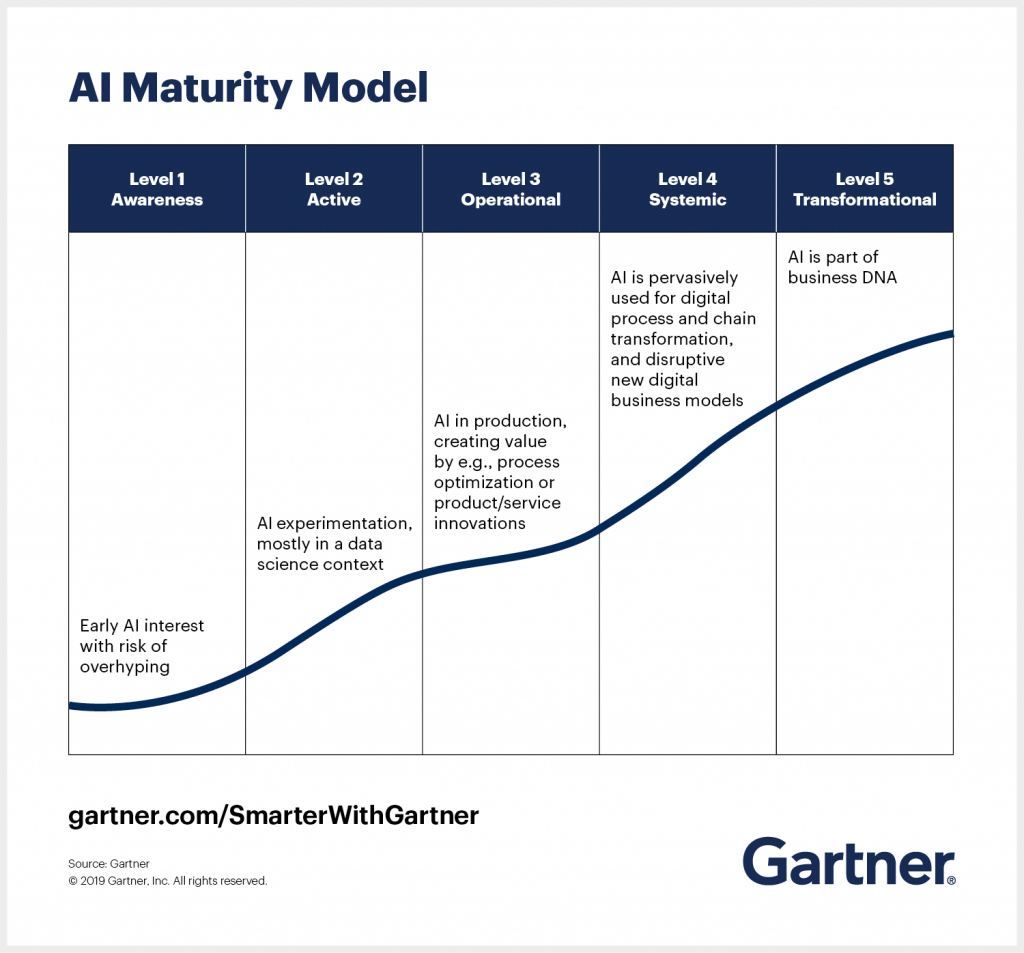
The CIO’s Guide to Artificial Intelligence, Gartner (2019)
2. Organizations will take a more unified approach to applying AI.
This year, organizations will advance how they operate and sustain machine learning models in production. CloudFactory is working with many teams in the later stages of model development, including evaluation, tuning, and testing. We also provide the humans in the loop for production environments.
Some teams use active learning to automatically and intelligently sample data for annotation (e.g., based on low confidence scores) and process the exceptions in real time to improve the model. In most cases, however, disconnected teams hold responsibility for different parts of the process. For example, the R&D team may have built the model but the operations team is running it, and there are manual processes between the two teams.
In 2021, we expect more teams to evolve from a siloed approach to a more unified approach for model development and production processes. More companies will experience the significant advantages of having a unified system and workforce that can annotate data and augment models across the full AI lifecycle, with continuous learning.
3. AI will be used to solve more meaningful problems.
The world will not see generalized AI for a good while. But we don’t need more science fiction movies, we need more problems solved, with the right blend of machine and human intelligence. The next 12 months will see more focus on the application of ML to solve meaningful, real-world problems.
Two factors are driving this: where we are on the AI adoption curve and the pandemic that has brought urgency and focus to solving real problems, from finding a COVID-19 vaccine to contactless food delivery.
Zipline deployed drones and used computer vision to deliver life-saving supplies to Rwanda and Ghana, replacing inconsistent daily deliveries by ground vehicles. At first, hospital staff in those countries saw futuristic drones dropping packages out of the sky. Now, they look at their watches after a drone delivery and ask, “Why was it 30 seconds late?”
When AI solves an urgent problem, we begin to rely on it. That means it must be refreshed with real-world data to ensure high performance and positive outcomes. Humans in the loop will continue to play an important role in training machine learning models and maintaining them in production so they can deliver high performance over the long term.
4. Access to data will increase.
AI is all about data, and 2021 will bring shifts in the availability of data. First, there will continue to be more paid and open source datasets available. These can be great for research, proof of concepts, and getting started. As teams begin to scale their AI projects, custom data collection becomes a key part of the challenge. This was the problem when the pandemic stopped or slowed data collection. We have seen our clients find ways to restart data collection, and some are making up for lost time by increasing their team with our ready workforce.
As enterprises have prioritized digital transformation and automation during the pandemic, many realized they were not capturing or storing their data in a way that it could be leveraged. That changed in the last half of 2020, when many enterprises changed their processes to collect, store, and structure new data so it can be useful later.
With more data available every day, it’s important to have a strategy for collecting and annotating data. We love sharing best practices for how to progress from a reactive approach to data collection and annotation to a more strategic one, which can stretch budgets further, speed time to market, and produce better performing models.
5. More teams will combine data sources to increase model performance.
Not only will access to data increase, but more projects are combining data sources in the same way humans combine the input of our five senses into a cohesive product. This is called multimodal learning and tech teams are fusing senses together to take their models to the next level of intelligence.
This has been most common in the area of autonomous vehicles (AV) with multiple sensors such as cameras and lidar being combined through sensor fusion in the labelling process. This same approach is being applied to more and more use cases, partially driven by the innovation and lower cost of sensors. Even in AV there is movement to incorporate visual and audio data from inside the vehicle to provide more context.
There are a lot of challenges in collecting real world data such as drivers getting angry (capturing the actual audio and video) and then the data pipelines, the labeling tools, and labeling process all become more complex. Multimodal sensor fusion is a natural next step for artificial intelligence and as many AI teams and programs mature this year we will definitely see more of it.
6. The trend toward data transparency, responsibility, and accountability will increase.
We will continue to see a focus on governance and ethics across the AI supply chain. No longer does the end justify the means in building a dataset to train a model. Where the data comes from, data ownership and rights, privacy preservation, and the people who access and label data will be more important than ever for organizations that are building mature AI programs.
At CloudFactory, we look forward to the tough questions we get from our clients about who is working with their data. We love to talk about our amazing data analysts, and we are proud of their training, skills, and dedication. They are the reason we do what we do!
No longer can data scientists turn a blind eye to the hidden ghost work that was synonymous with the last decade of this AI boom. Let’s make a step forward in 2021 by knowing, valuing, and celebrating the humans in the loop who train and maintain the AI that is changing our world for the better.
What are your data and AI predictions for 2021? We’d love to hear from you on LinkedIn or Twitter. We wish you a prosperous, healthy new year!