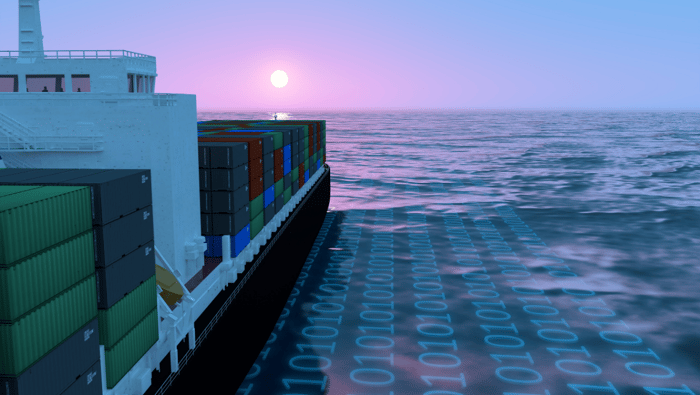
We are swimming in an ocean of data. The ways we gather and leverage it are fueling innovation in artificial intelligence (AI) and machine learning, even in a pandemic. But there’s a catch: data must be thoughtfully cleaned and processed for an AI concept to come to life.
Some organizations jump right in with visions of leveraging game-changing AI technology. However, many are quickly overwhelmed by the vast amount of data processing required to train, tune, and move to production AI models for predictive analytics, computer vision, or natural language processing (NLP).
It’s a common problem. According to Forrester, “most enterprise AI models don't make it into production, and many stall at the pilot or proof-of-concept phase, even when they show value.” The report cites data quality and talent scarcity as factors that are particularly challenging and serve as a reality check for teams building AI solutions.
Quality data begins with data labeling. To create, validate, and maintain production for high-performing machine learning models requires trusted, reliable data - and a lot of it.
It takes time and skilled people to gather, annotate, and quality-check the massive amounts of data required for machine learning. For example, to develop computer vision for an autonomous vehicle requires labeling countless frames of video to teach the algorithm to “see” objects such as people, signs, trees, and vehicles. For every one hour of video, there are hundreds of hours of labeling work to be done.
Getting access to a large number of workers who can deliver quality data labeling can be a challenge, especially when speed is important. Most AI project teams begin the work in-house, which they find doesn’t scale.
Crowdsourcing can provide access to large pools of workers but they will be anonymous, which makes accountability and quality a challenge.
Business process outsourcing operations (BPOs) are rigid and rely on facilities to deliver the work. The COVID-19 pandemic has shuttered the doors of many traditional BPOs who couldn’t distribute their workforce remotely, leaving their clients without support for the ongoing data labeling needs.
The managed-team approach
At CloudFactory, we’ve learned it takes a strategic combination of people, process, and technology to address talent scarcity by scaling large teams of workers to achieve the highest quality data labeling.
- People: Workers who are known, carefully selected, and valued for their strengths deliver high quality. Workers should be applied to tasks based on their skills and interests. Working in small, managed teams, they can share best practices and train new team members.
- Process: Change is a constant with AI projects, so processes must leave room for tasks to evolve and change. Workers must understand how the data will be used and understand how to deal with edge cases. In managed teams, workers’ knowledge of the use case and task requirements increases over time, which improves quality.
- Technology provides access to a large number of workers and facilitates direct communication with a leader on the ground. It also eases scaling the work up or down based on what is needed.
To label an ocean of data takes a great workforce partner who understands the challenges of AI development and the importance of data labeling to its success. To explore how a dedicated, managed team can improve quality and worker availability for your AI project, contact us today.
Data Labeling Workforce Strategy AI & Machine Learning Data Cleansing