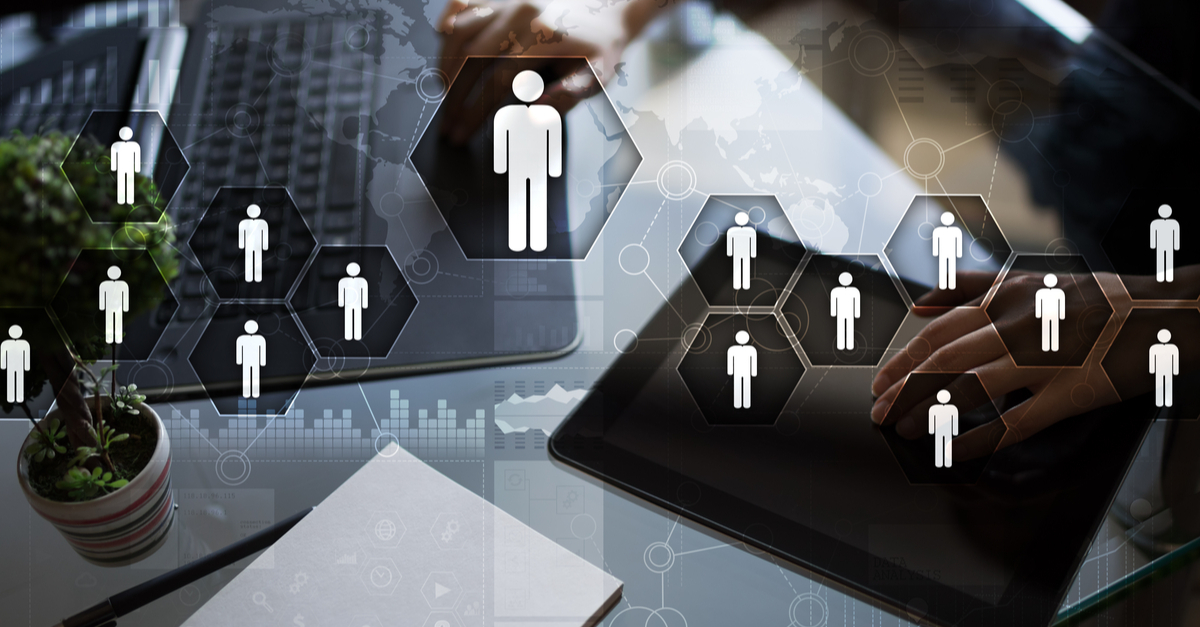
The importance of people in AI development cannot be understated. The humans in the loop are necessary to create machine learning models, and they can be involved in everything from data labeling to automation exception processing. When you strategically apply people, process, and technology, you can create higher performing AI systems.
Managing data labeling in-house is challenging so many innovators outsource the work. However, you must choose wisely when selecting your workforce.
In a recent Hype Cycle for AI, Gartner recommends you “ensure the provider you choose has methods to test their pool of knowledge workers for domain expertise and measures around accuracy and quality.1”
How can you determine if a data labeling service provides workers who will deliver quality work? This week we’ll explore how hiring, vetting, and context impact data quality. Stay tuned for next week’s post about communication and quality assurance processes.
Not Everyone Can Be a Data Labeler
You have a few workforce options, and each one hires and trains workers differently. Crowdsourcing is often touted as an affordable and scalable solution for data labeling for AI, but it comes with serious caveats.
The main problems with crowdsourcing are that workers are anonymous and are often not sufficiently trained. Crowdsourcing can distribute data labeling tasks to independent workers nearly anywhere in the world, so there’s no way to vet their expertise or validate their skills until your work is completed, which can affect quality.
Data quality might not be a serious problem in more simple, lower-risk AI projects, such as those designed for entertainment (although, does anyone really want their brand associated with an AI snafu?). However, if you’re developing an AI model where inaccurate predictions can affect people’s lives, such as AI applications for healthcare, autonomous vehicles, or predictive maintenance of industrial control systems, data quality is quite a different matter. Crowdsourcing leaves little room for accountability and can result in data quality issues that can create negative outcomes.
It’s important to choose an outsourcing provider with an in-depth worker vetting and selection process that pairs well-trained labelers with the right projects. That’s not going to happen with crowdsourcing, and it isn’t easily scalable with in-house data analyst teams. When data quality matters, your best choice is a managed workforce that serves as an extension of your own team.
Context Matters
There’s far more to data labeling than simply drawing bounding boxes around objects. The people tasked with these jobs must also have an understanding of the business objectives and how the work they are doing will be used in real life. That context provides workers the ability to make subjective decisions based on nuances that can affect the quality of the annotations.
For example, let’s say you’re developing an AI system to detect signs of disease in crops. In this case, the people who are annotating your images and videos need to be able to identify and distinguish between things like crop types, growth stages, and regional or seasonal diseases. If you’re like the agriculture technology company Hummingbird, you have an agronomist who is an expert in these matters but their time is too expensive to use on quality control for data labeling. Instead, Hummingbird tapped a managed workforce with known, vetted workers. Their agronomist wrote the data labeling requirements, which the data analyst teams use to annotate accurately and train new team members.
Training is an essential part of delivering high-quality data labeling work. Moreover, training needs to be tailored to the individual needs of the client. Every AI project is different, hence the need for targeted onboarding and ongoing training when building a managed team of expert data labelers.
Choose Wisely
AI project development depends on human expertise. That’s why a provider’s hiring and vetting process must be carefully considered when you outsource data labeling. The team must consist of labelers who understand the nuances of your project and can make subjective decisions with high accuracy. Choose an annotation partner that invests in training and nurturing talent, and your odds of receiving high-quality data increase exponentially.
Outsourcing Workforce Strategy ML Models AI & Machine Learning