Healthcare organizations are at the forefront of using AI to foster medical breakthroughs. AI promises to improve patient care, help make more accurate medical decisions, and lower costs. One specific area of AI that healthcare organizations are eagerly tapping into is computer vision.
Computer vision, a field of AI that enables systems to derive meaningful information from digital images and videos, is helping medical professionals gain valuable insights such as noticing patterns that might be invisible to the human eye and keeping track of surgical supplies during procedures. As a result of the increasing demand to apply computer vision to the medical field, the Global Computer Vision in Healthcare Market is expected to reach $416 billion by 2025.
How To Handle The Demand For Computer Vision in Healthcare
As you can imagine, the U.S. produces a massive amount of medical images. It’s estimated that 40 million MRI scans, 80 million CT scans, and 150 million X-rays are performed on patients every year in the United States. For computer vision to aid in diagnoses, millions of medical images must be expertly labeled to train, validate, and test machine learning models. This labeling takes a significant amount of expertise and medical knowledge to execute correctly.
Complicating the process is that the medical industry is not immune to the current global skills shortage. According to the Association of American Medical Colleges, the U.S. faces a shortage of between 46,900 and 121,900 physicians by 2032.
Additionally, radiologists are among the most in-demand specialists in the U.S. While radiologists play a crucial role in analyzing medical images at the point of care, it is impractical to scale data labeling projects to train computer vision models solely relying on radiologists. They simply don’t have the bandwidth to review and label countless images. And the same logic applies to other disciplines within the healthcare field — they have many other things they need to do and focus on, and data annotation is typically not the best use of their time.
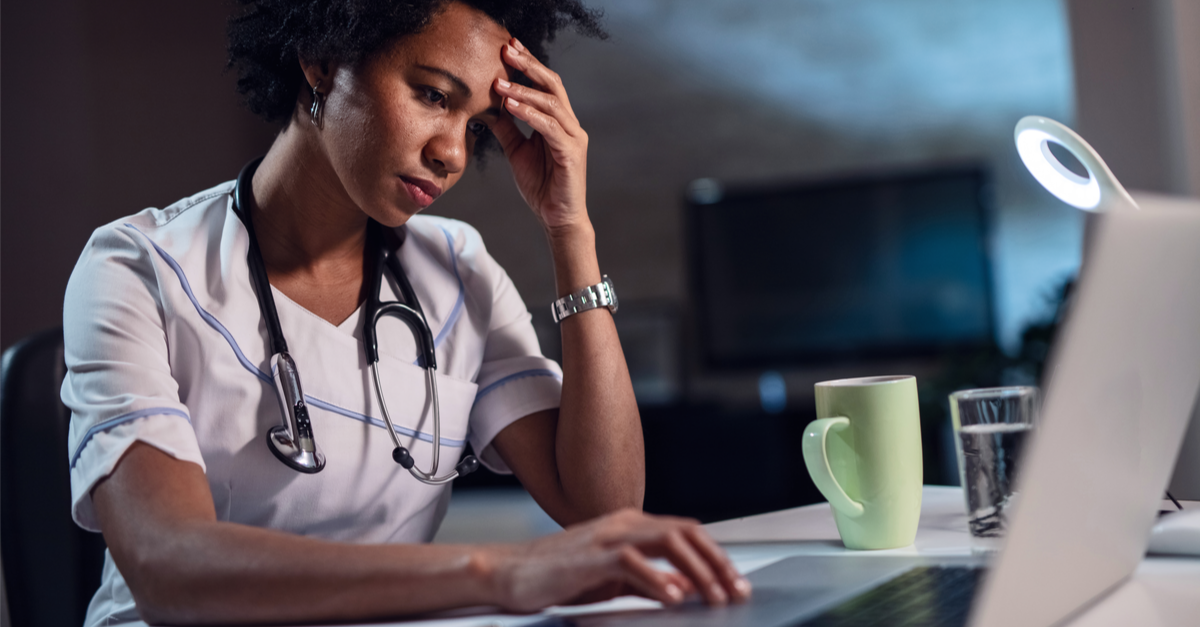
Take The Burden Off Your Medical Staff: It's Time for a Data Annotation Provider
The solution is finding a data annotation provider who understands straightforward and complex data annotation techniques. For example, image annotation, also called image classification or tagging, could be as simple as identifying an anatomical or cellular structure like a lung or a cell wall. However, a more complex image annotation task may involve identifying, counting, or tracking multiple objects or areas in an image, zooming in on an irregular MRI scan image, or the knowledge to manage potential edge cases.
While the complexity of your annotation project will vary, the right annotation provider needs to be confident about which image annotation method will yield the desired outcomes.
How Do Medical Professionals and Data Annotation Providers Work Together?
Medical professionals remain an essential role in this process, working alongside trained data annotation teams to scale the process affordably and efficiently using a variety of tools and approaches. One approach is conducting video training courses and one-on-one sessions with team members. Another is to identify and transfer best practices learned in one data labeling project back to teams working on subsequent projects.
Best Practices When Considering a Data Annotation Provider
Here are five essential best practices when considering a data annotation provider for your medical AI project:
1. Analysis
A data annotation provider should perform a thorough and complimentary expert analysis of your project requirements and deliver a robust playbook with task feedback, QA workflow recommendations, and ideal workforce skills during the proposal process. The goal is to ensure mutual agreement on the project scope and cost.
2. Seed
Look for a provider that chooses a cross-functional team of highly-skilled data annotators. Then, using agile methods, they can prototype your tasks and design a custom training program to onboard additional data annotators quickly and ensure long-term efficiency, productivity, and high-quality work.
3. Training
A reputable provider will assemble a dedicated and skilled team of data annotators. The team members will receive training using a combination of real-time instruction and coursework to ensure understanding. Training topics may include tooling and task guidelines and initial requirements for accuracy and throughput.
4. Production
Quality data annotation providers will initially focus on precision, and once proficiency and high-quality work are demonstrated, they will increase throughput. A dedicated project manager should continue to coach and manage team members during this process, keeping you apprised of progress through a continuous feedback loop.
5. Scale
Annotation partners should adjust for capacity and have flexible subscription models to meet your needs.
In addition to the best practices listed above, here are three other things to consider when evaluating data annotation providers for your medical AI project:
1. Assuring Quality in Medical AI
Look for labeling providers with a strong record of delivering high-quality data. Certifications like ISO-9001 demonstrate a commitment to quality management. Ask questions about their quality assurance and quality control processes. Proven, established processes will help ensure accuracy is a priority for the labelers.
2. Scaling Medical Image Annotation
Labeling providers should have experience labeling large, complex healthcare datasets in a variety of use cases. It's also easier for providers to scale additional projects if they have a team of experienced medical labelers to call upon.
3. Ensuring Data Security
Data security should be a priority for healthcare labeling providers. Look for security offerings beyond HIPAA, such as GDPR, Soc2, and ISO-9001. Superior annotation vendors will also work with clients to uncover and accommodate unique healthcare security needs.
How CloudFactory Can Help
CloudFactory works closely with your team to understand complex medical AI use cases. In addition, we’ll work with your team to develop rigorous training materials that help our data analysts execute highly technical medical image data labeling, annotation, and quality assurance tasks.
CloudFactory’s medical image data analysts go through multi-level assessments to understand that visual data annotation is fundamentally different from tasks like text transcription. We give our data analysts test data, monitor data quality closely, and identify which workers develop competency on business rules and labeling details. As data analysts repeat tasks over time, they gain efficiency, and the labeling and medical annotation work become easier with higher-quality and faster throughput.
Why is CloudFactory your best choice for medical AI data annotation?
Quality and scalability are difficult to achieve, which is why CloudFactory’s managed workforce is the right balance for medical AI data annotation. We’ve successfully annotated millions of medical images for a range of clients working in medical diagnostics, robotics, and research. Learn how our managed workforce supports medical AI, including labeling diagnostic and clinical imaging here.
Data Labeling Workforce Strategy Computer Vision Healthcare AI & Machine Learning