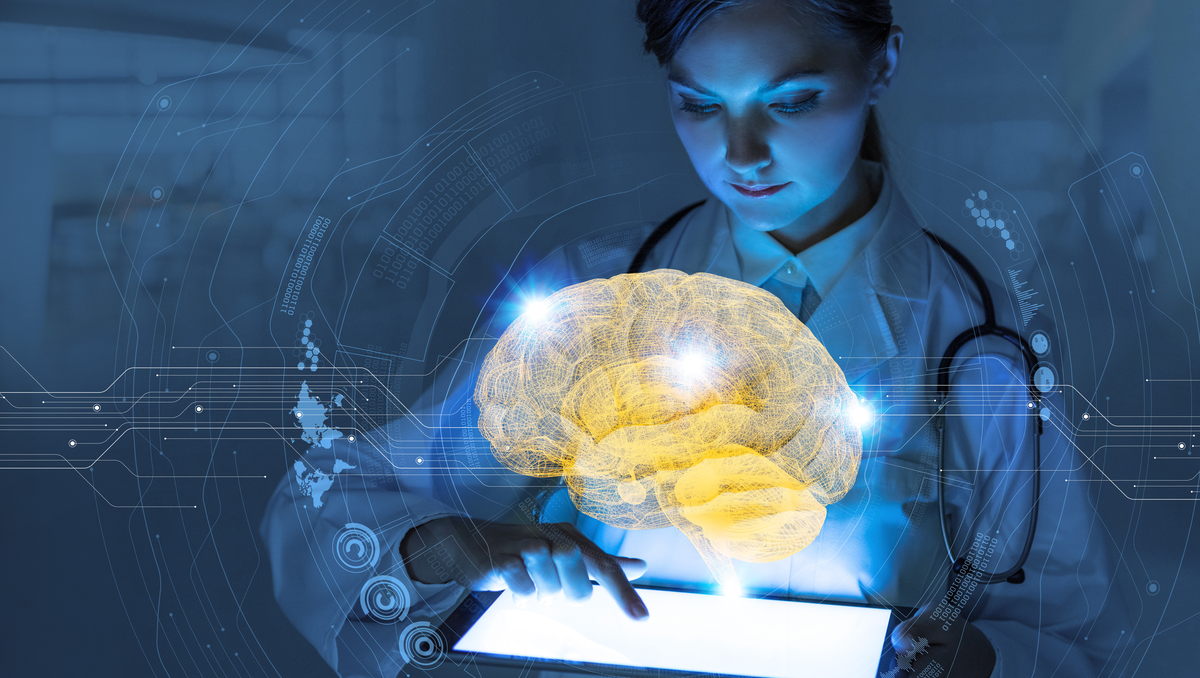
Artificial intelligence (AI) seeks to mimic human cognitive functions at machine speed, rather than relying on preprogrammed behaviors alone. The rapid pace of development in the AI sector, empowered by massive funding by investors, is set to revolutionize healthcare and many other industries.
AI models may be applied to various types of healthcare data, including both structured and unstructured forms. For example, machine learning models can translate large sets of structured data into actionable insights that help practitioners make smarter decisions in less time, leading to better patient outcomes. More sophisticated models go even further to the point of assisting with complex medical processes like drug discovery, medical diagnoses, and precision medicine.
Yet despite the enormous benefits of AI in healthcare, substantial challenges remain. AI was born out of the need to maximize the untapped value in increasingly large data sets that are too big for manual interpretation. Bringing medical AI to life requires extensive preparation, training, and a workforce at all stages in the cycle of continuous improvement.
Here is what that process looks like with humans in the loop (HITL):
Stage 1: Design and Build
Data is the fuel for building and training an AI model, and it is required in enormous amounts. The more data available, the more accurate the model, and there can never be too much. In an area like medical AI, the need for accuracy is particularly great, and the scope for error is minimal. Moreover, there are additional challenges in acquiring healthcare data in the first place, due to privacy regulations like HIPAA. As such, the data must first be anonymized by removing all personally identifiable information before using it to train the model.
The first stage of AI model development is acquiring the data in the first place. Healthcare data is often visual, existing in the form of MRI scans and X-rays, and there are also many applications for NLP, all of which require specialized domain knowledge to interpret. That is why a human workforce is essential for preparing the data and teaching the model how to interpret it. For example, if a medical AI is to determine the progress of respiratory ailments like COVID-19, then it must be trained with large numbers of chest X-rays. During the design and building stages, these X-rays must be correctly annotated to teach the machine how to interpret them. Eventually, once the machine has been given enough accurate training material, it should be able to identify medical conditions on its own when scanning X-ray images.
Deploying humans in the loop (HITL) is essential during this process since the data must be manually collected, cleaned, and annotated. This ensures the data is relevant, up to date, and of a high enough level of quality to be useful in training the model.
Stage 2: Deploy and Operationalize
After the initial training phase, it is time to put the model to the test. This is where most failures occur, and it is only to be expected. In fact, 96% of failures occur during and after this phase, hence the need for ongoing testing and refinement. Because issues with data quality can mean the difference between sickness and health in medical AI applications, quality control is critical for ensuring the model is ready for use in real-world situations.
During this stage of AI model development, we can use auto-labeling to determine how well the model understands the data. At this point, it is inevitable that the model will make mistakes, thus pointing to the need for further training. This is why, to ensure quality control, we also need to use a human workforce to constantly check for any errors or inconsistencies. With the optimal combination of people and technology, it is possible to alleviate some of the burdens of scale, while reducing costs.
The synthesis between people and technology streamlines the process to create a continuous feedback loop. For example, if the AI reports a low confidence level when looking for a specific ailment in an MRI scan or X-ray, it will flag it for manual review. In cases where this continues to happen, it indicates a need for retraining. At this point, development can move onto the refinement and optimization stage.
Stage 3: Refine and Optimize
No AI will ever be perfect. That said, through constant refinement and optimization, the model can become every bit as effective as human decision-makers. Since AI also works at machine speed and makes decisions based on facts rather than emotions, it also negates the risk of human error and augments the abilities of time-strapped healthcare staff and research teams.
As we have witnessed from the unprecedented development of the COVID-19 pandemic, the world of healthcare and medicine is often unpredictable. Three or four new viruses that affect humans are detected every year alone, and biological conditions are every bit as changeable as the environment. Because of this, medical AI used to assist operations like diagnosis and drug discovery must be constantly refreshed and optimized.
When medical AI detects a condition it has never seen before, an exception occurs that must be handled manually. If too many exceptions are allowed to occur without undergoing manual review, then the model can regress in terms of accuracy and effectiveness. As is the case with people, it can be difficult to shed these bad habits if they become too ingrained. This concept is known as AI drift and is caused by factors like the proliferation of edge cases or a lack of consistent quality control over the data used to train it.
A managed workforce familiar with the context and goals of the model is essential to overcoming these challenges by overseeing a cycle of continuous improvement. By contrast, popular methods like crowdsourcing or working with gig workers make it harder to maintain the high quality of data preparation and annotation needed to keep refining the model. In other words, a managed workforce facilitates an optimal collaboration between people and machines so that, together, they can get ahead of illness and drive better healthcare outcomes.
Learn more about how our managed workforce and security offerings can help you meet the challenges of scale in medical data annotation.
Workforce Strategy Computer Vision Healthcare AI & Machine Learning