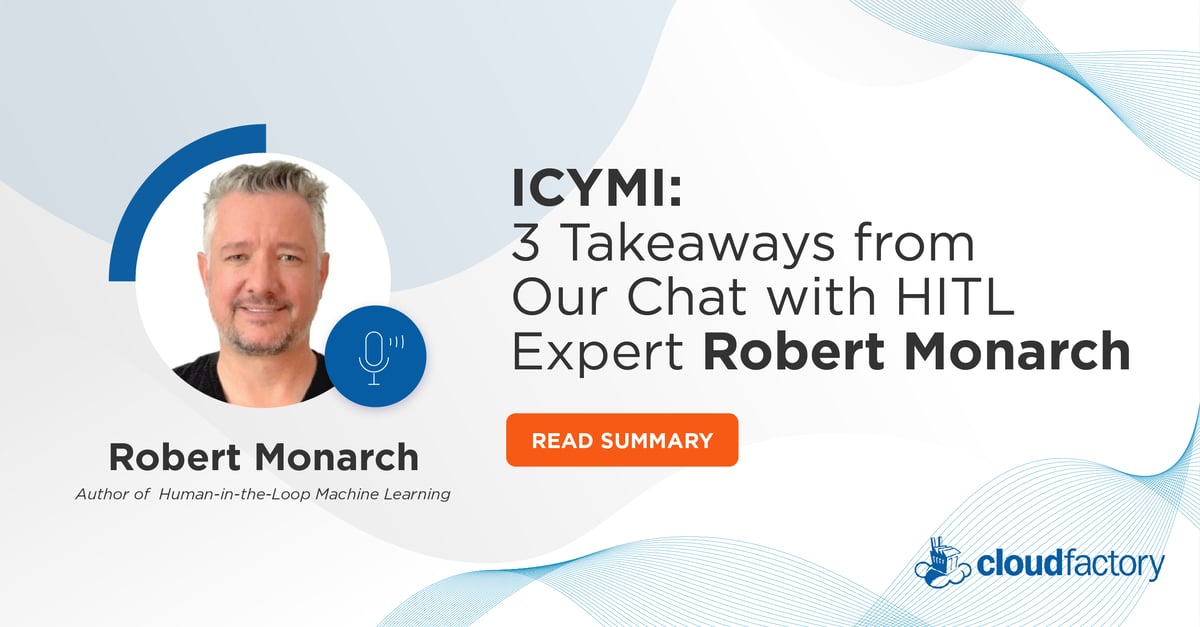
In our latest LinkedIn Live event, our Chief Data Science Advisor, Keith McCormick, spoke with Human in the Loop Expert and Author, Robert Monarch. We explored what it takes to combine human and machine intelligence in the most effective way. Here are some key insights from the session.
The development of machine learning models traditionally relies on vast amounts of structured data for training. However, the vast majority of the world’s data is unstructured, existing in just about every form imaginable from news articles to social media posts to images and videos. This data is among the most valuable resources of all, yet it remains largely untapped. But how do we teach a machine to fully understand all the nuances of content created by and for a human audience, and what does it take to build a workforce that can achieve such a feat at scale?
“We’re seeing a big trend towards AI supporting human processes. This could be something as simple as autocomplete, or something more sophisticated, like helping a medical professional interpret an X-ray. In the latter case, you also have to consider the human-to-computer interaction and user experience principles, so that human and machine intelligence can be combined in the most effective way.”
—Robert Monarch
1. Choosing the Right Workforce
There has long been a disconnect between data annotation and AI model training. For too long, data annotation has been viewed as a tedious and repetitive task that requires minimal experience or expertise. The truth is, however, that an AI model will only ever be as effective as the processes used to collect and annotate the data required to train it. This requires—in a lot of cases—domain-specific training or knowledge and close collaboration between data scientists and data annotation teams. There are three ways to approach this workforce challenge:
- Organizations seeking ways to automate or semi-automate routine processes usually rely on in-house teams, despite the lack of scalability and higher costs.
- Managed workforces are the second-most popular option and the fastest growing, as they offer scalability and seamless alignment with in-house teams.
- Crowdsourcing, while once the most popular approach, is falling out of favor due to the lack of oversight, consistency, and quality control.
With the right approach, it is possible to implement a feedback loop that continuously improves the model. For most projects, the best approach is to use a managed workforce in which each worker enjoys job security, and the team works closely with your own to help you achieve your goals. These teams are also more likely to work long enough on specific projects and industry sectors to develop critical domain knowledge. For example, if a machine learning model is to help medical professionals correctly understand X-ray images, then data annotation teams will need to have familiarity with that industry and use case.
2. The Role of Expertise in Data Annotation
Another way of looking at the feedback loop is to distinguish between crowdsourcing, human-in-the-loop (HITL), and expert-in-the-loop. Different projects require varying levels of expertise, and it often makes sense to bring in a dedicated professional with domain knowledge. Those who have acquired such domain knowledge often have years of experience to the point they develop an intuition when it comes to data annotation. To that end, they might be even better equipped than a highly skilled data scientist to recognize things like edge cases, linguistic differences, and biases.
Data annotation is a science in itself, and one that should be taken seriously for its crucial role in the global information economy. For example, a managed data annotation workforce can bring a diverse range of life experiences and personality traits into the mix, which makes them more adept at eliminating AI bias to develop more viable models. Data scientists, on the other hand, tend to have more technically oriented responsibilities, such as modelling, analytics, and statistics. In the case of human-in-the-loop, both play extremely important roles.
One of the most common misconceptions around data annotation is that it only requires soft skills, and that there is really no such thing as advanced annotation. This is perhaps the main reason why most courses on machine learning focus heavily on algorithms, often at the expense of human-to-computer interaction. Despite this, most AI solutions in use today depend on human feedback to maintain their accuracy and usefulness.
Maintaining quality control throughout the AI project lifecycle simply cannot be done without a suitable data annotation workforce. Quality control requires a diverse team to avoid bias and prevent narrow subjective views from dominating the AI model. Such a team cannot be built if all the focus is on data science and algorithms. After all, annotation is easy to underestimate, and being a PhD student, for example, does not necessarily make someone ready for the job.
3. Managing Humans-in-the-Loop
Managing human-in-the-loop machine learning projects requires an optimal blend of people, process, and technology. Mixing and matching resources can help optimize cycle times and cost control, not least because every project has different needs and goals.
One of the most effective strategies for managing data annotation is the hierarchical approach. For example, the first stage of data annotation work might be a simple binary classification, as in the famous hotdog/not hotdog case. However, many projects are vastly more complex, and require much more granular control over data annotation. In the case of labeling text data for sentiment analysis, one might begin by classifying whether or not a phrase is even relevant for labeling in the first place. Then, a deeper analysis might explore how many single minor edits it might take to completely change the meaning of the phrase. It is, after all, very easy to completely change the meaning of a phrase with a simple typo, at least insofar as a machine learning model is concerned.
In another example, annotating a model used to classify disaster-related messages from real disasters might start with a simple binary classification to determine whether a given headline is related to a real-world disaster or not. Then, it may be necessary to look at other factors that might be relevant, such as the location the headline pertains to, the information it offers, and whether it includes any subjective biases. The latter can be especially problematic, since you will probably want to avoid imprinting bias into the machine learning model and instead have it recognize and understand the objective views of a news report.
Bridging the Gap with a Managed Workforce
Overcoming the disconnect between data annotation and AI model training requires rethinking the overall process. Above all, data annotation teams should work closely with data science teams to ensure a seamless collaboration, as well as maintain oversight of quality control. The best way to achieve this, for most projects, is to hire a managed workforce where teams have the necessary domain knowledge to get the job done to a high standard.
Learn even more about what it takes to combine human intelligence and machine learning effectively by watching the full on-demand video of the chat between Keith and Robert. To participate in future events, please follow CloudFactory on LinkedIn.
Data Labeling Workforce Strategy ML Models AI & Machine Learning Events