An overview of MLOps field, its similarities & differences compared to DevOps, & how it can help organizations navigate common issues.
CloudFactory Blog
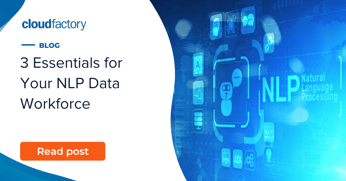
Natural language processing (NLP) is a fast-growing AI technology, but data labeling for NLP is complex. Here is what to look for in an NLP data workforce.
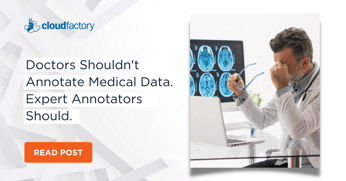
Doctor shortages are impacting healthcare innovators’ ability to label data for computer vision solutions. Outsourcing the work will help.
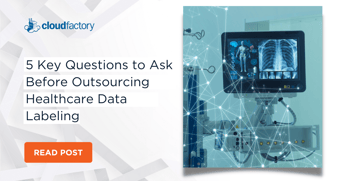
Computer vision improves patient care, streamlines medical decisions, and lowers costs. Ask these questions before outsourcing healthcare data annotation.
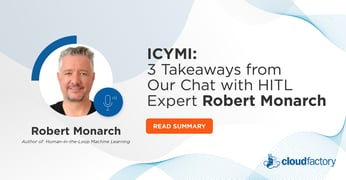
Learn 3 key takeaways from our latest LinkedIn Live event where we explored what it takes to combine human and machine intelligence effectively.
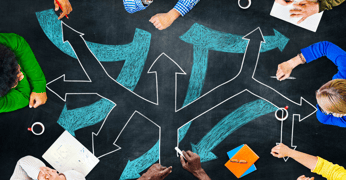
An incremental design approach to automation and machine learning affords strategic opportunities for choosing to route exceptions to machines or people.
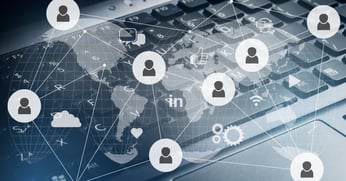
Data entry is a crucial part of any digital transformation project. Sometimes it makes more sense to outsource than to burden your own team.
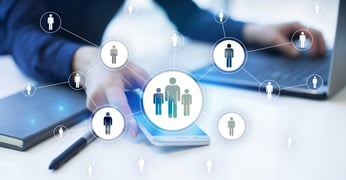
How can you determine if a data labeling service will deliver quality work? How they communicate and handle quality control are key indicators.
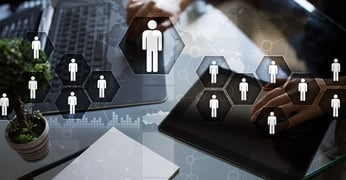
How can you determine if a data labeling service will deliver quality work? It starts with their vetting, hiring, and training processes.
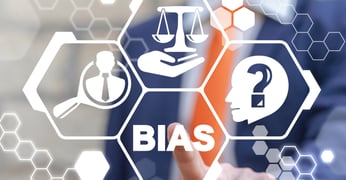
People have unconscious biases that affect hiring decisions. People also can hard-code their biases into an AI system. Humans in the loop can help.
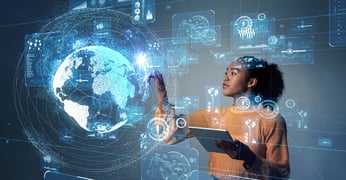
Humans play a critical role throughout the AI lifecycle, from data cleaning and labeling to quality control and automation monitoring.
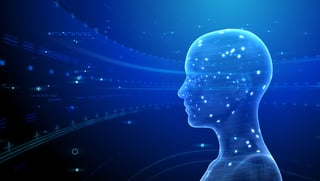
Developing ML models requires a lot of data and skilled people to work with it. Here’s our HITL approach for machine learning model development.
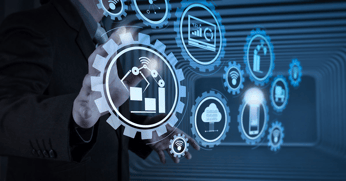
Supervised learning requires a lot of labeled data. Here’s what it takes to design a high-performance data labeling pipeline for machine learning.
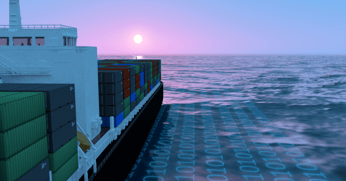
Even in uncertain times, you’re swimming in an ocean of data. How you are processing data that powers AI and use that data will determine the future of your business.
![Crowdsourced Workers vs. Managed Workers [Infographic]](https://www.cloudfactory.com/hs-fs/hubfs/04-blog-img/infographic-social-post-crowdsourced-vs-managed.png?width=346&height=181&name=infographic-social-post-crowdsourced-vs-managed.png)
Data scientists at Hivemind created 3 data labeling tasks and hired 2 teams to complete them. The differences in data accuracy, speed, and cost may surprise you.
![5 Qualities in Good Data Labeling Vendors [Infographic]](https://www.cloudfactory.com/hs-fs/hubfs/04-blog-img/cf-infographic-social-post-5-qualities.png?width=346&height=181&name=cf-infographic-social-post-5-qualities.png)
Not all outsourced data labeling partners are a good fit for every AI project. Here are 5 things you need to consider before, during, and after vendor evaluations.