Many companies are having to contend with new data security concerns associated with their employees accessing important data from home.
CloudFactory Blog
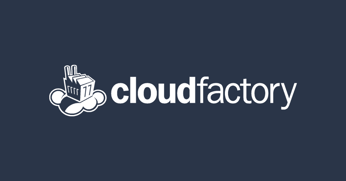
CloudFactory responds to the COVID-19 pandemic with remote access for workers, business continuity for clients, and community service.
![In-House vs. Managed Workforce Data Labeling Partner [Infographic]](https://www.cloudfactory.com/hs-fs/hubfs/04-blog-img/cf-infographic-social-post-inhouse-vs-managed-workforce.png?width=346&height=181&name=cf-infographic-social-post-inhouse-vs-managed-workforce.png)
It takes a lot of time and resources to prepare and label data. Learn why outsourcing the data preparation to a managed workforce partner is a good business decision.
![6 Ways Data Labeling Providers Put Your Data Quality At Risk [Infographic]](https://www.cloudfactory.com/hs-fs/hubfs/04-blog-img/cf-infographic-social-post-6-ways.png?width=346&height=181&name=cf-infographic-social-post-6-ways.png)
The level of data quality you'll receive from data labeling providers depends on several workforce, QA and tooling factors. Here are 6 ways some data labeling providers put your ...
![3 Signs Data Labeling Provider Delivers Quality Data [Infographic]](https://www.cloudfactory.com/hs-fs/hubfs/04-blog-img/cf-infographic-social-post-3_signs.png?width=346&height=181&name=cf-infographic-social-post-3_signs.png)
The people, processes, and tools used by outsourced data labeling partners make a big difference in final data quality. Here are 3 signs that you'll receive quality work from your ...
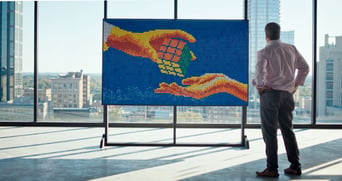
Achieving a high level of accuracy in data labeling is vital. This concept can be understood if we think about a mural of Rubik’s Cubes®.
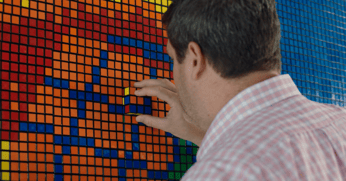
Any problem (like a Rubik’s cube®) is solvable with a documented process.
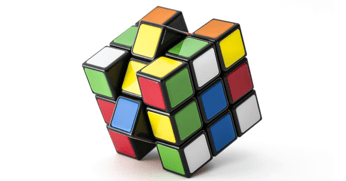
How solving a Rubik’s cube® is like labeling your unstructured data.
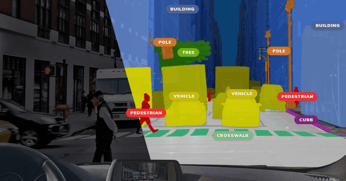
When you have massive data to label for machine learning, it makes sense to outsource it. But what happens when your data is sensitive, protected, or private? Here’s a quick ...
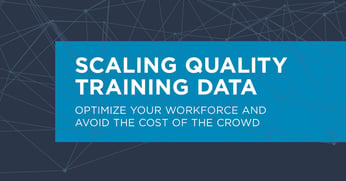
Crowdsourcing seems to offer a cheap option for training machine learning models, but it’s rarely as inexpensive as it seems. Here are some of the hidden costs of the crowd.
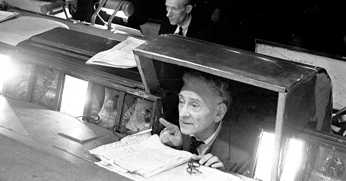
Humans are the builders and the trainers of every AI technology, and humans will be the cornerstone of work for a long time to come.
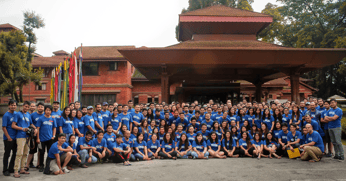
How CloudFactory is different from crowdsourcing platforms like Amazon Mechanical Turk (mTurk) to handle critical business processes that need to be done right.