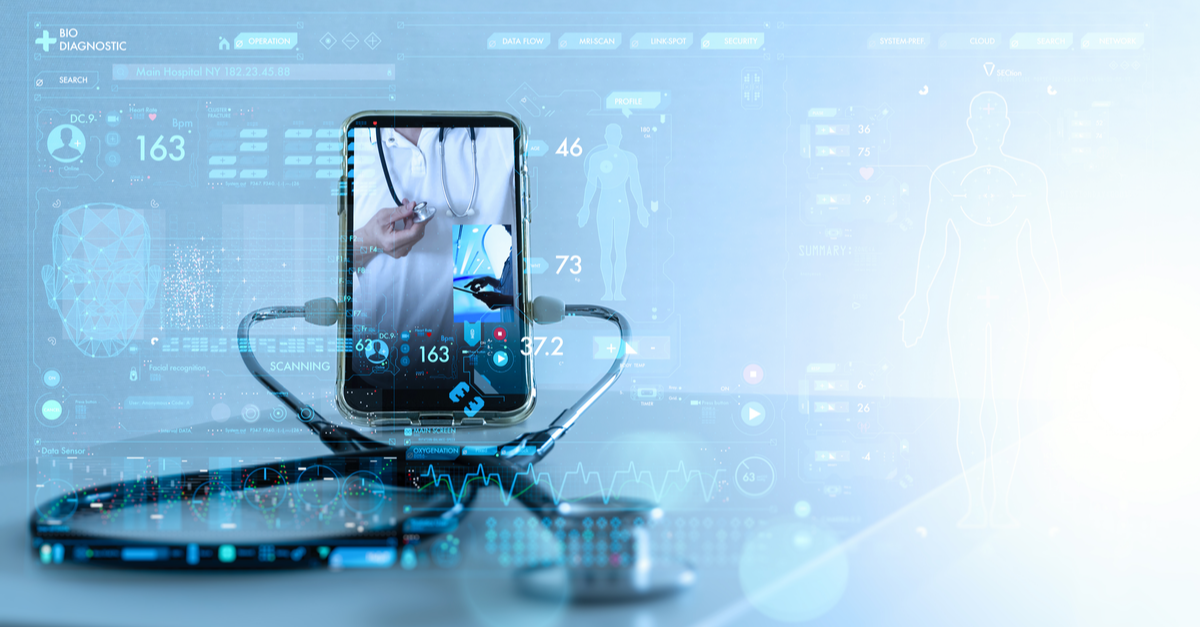
The evolution of healthcare is one of the greatest success stories of modern times. But, as the ongoing coronavirus pandemic and the rising threat of antibiotic-resistant drugs have shown, there is always room for improvement. As life expectancies increase, healthcare services face constantly growing demand. Rising costs and a need for more healthcare workers are making it harder to meet those needs and improve outcomes.
Despite the unstoppable forces driving demand, new technologies like artificial intelligence (AI) promise to make healthcare more scalable and sustainable. With investors pouring $4 billion into healthcare AI startups in 2019 alone, it is safe to say the industry is undergoing a radical transformation. AI is becoming instrumental in speeding up operations like drug discovery and diagnoses, while robots will assist in complicated surgeries.
Here are some of the most exciting applications for medical AI:
1. Medical diagnosis
Applying AI to medical diagnoses can greatly augment the capabilities of medical teams by automating routine diagnostic operations, minimizing human error, and saving time. The technology has now reached a level where, in applications like cancer detection, it can perform at the same level as trained and licensed radiologists. Because AI works at machine speed, the automated analysis of medical images like MRI scans and X-rays happens much faster, allowing doctors to diagnose diseases in less time.
AI-powered diagnosis is also helping us garner a better understanding of COVID-19. V7 Labs is one company creating an annotated dataset so researchers can better understand how COVID-19 impacts the lungs in the longer term.
2. Robotic surgery
Performing surgical tasks requires an immense degree of care and accuracy. Whether routine or specialized, these often time-consuming operations can quickly become a massive burden, and overworked surgeons can introduce an increased risk of human error, with potentially catastrophic consequences.
While robots probably won’t be fully replacing surgeons any time soon, they can complement surgeons’ capabilities with better imaging and greater precision in factors like speed, depth, and trajectory. Patients benefit too with quicker healing times and less pain and scarring from robotic-assisted surgeries that are becoming more common.
However, because surgery is often nuanced and requires adaptability, it cannot always be automated according to pre-programmed behaviors. There is a clear and growing need for AI that uses deep-learning data to supervise the process and augment surgical capabilities.
3. Virtual nursing
AI-powered virtual assistants such as Amazon Alexa and Google Assistant have already been a part of our lives for some years, but recent advancements in technology are enabling a similar concept in healthcare and other sectors. Many nursing routines are repetitive and do not necessarily require a human touch, in which case they can and should be automated to free up time for nurses to focus on things that only they can do.
Virtual nursing assistants and chatbots are important factors in the changing healthcare equation. They can guide patients through various self-care routines, offer medical advice, and automate routine operations like appointment scheduling and patient admission and discharge—especially useful for remote patients.
4. Drug discovery
Pharmaceutical development is incredibly expensive, taking years or even decades to develop new drugs and test them for efficacy and safety. One of the main reasons for these long time spans is that it often requires a monumental effort to analyze vast data sets. On the other hand, thanks to the digitization of health records and other medical documents and archives, AI and automation technologies can be used to comb through the data, uncovering relevant insights at a much faster rate.
For example, global pharmaceuticals company and developer of one of the leading COVID-19 vaccines, Pfizer, uses the IBM Watson machine-learning platform to change drug discovery for the better, making it quicker, cheaper, and more effective.
5. Precision medicine
The goal of precision medicine is to build and optimize diagnostic and treatment pathways and develop more accurate prognoses. Clinicians have the opportunity to act more quickly with improved outcomes, detect the early onset of cancers and other diseases, and develop customized therapies and personalized medicine.
While a revolutionary advance in healthcare, such an approach relies on AI to analyze ever-increasing amounts of unstructured and unlabeled data sets to capture and understand key variables in everything from environmental factors to genetics to a patient’s previous medical history.
6. Administrative tasks
The U.S. spends 17% of its GDP on healthcare, outspending most other developed countries on a per capita basis. Much of this is due to burdensome administrative tasks and red tape, which don’t always directly lead to better healthcare outcomes. For example, in the U.S., almost half of healthcare funds are spent on administration.
By applying intelligent automation to many of these routine operations, costs could be reduced by improving efficiency, leaving more funds available to support affordable healthcare or further advancements in the industry. After all, AI can help overcome the challenges of scale, allowing hospitals to make better use of their resources.
How can a managed workforce help?
Despite these amazing developments and breakthroughs, scaling AI in healthcare remains a big challenge. Data sets need to be curated, cleansed, and annotated accurately. These labor-intensive tasks make clear the need for outsourcing. However, given regulatory concerns, the need for accuracy, and the question about whether only healthcare experts should do the work, these are not the sort of processes healthcare organizations should outsource to just anyone.
In fact, the CloudFactory team worked with one medical AI company to label medical images at scale, so it could develop its predictive advice algorithms to help practitioners garner a better understanding of health issues to aid in preventative care.
This is where the value lies in working with a reputable and experienced managed workforce to scale AI model development without compromising on quality.
Learn more about how our managed workforce can help you meet the challenges of scale in medical data annotation.