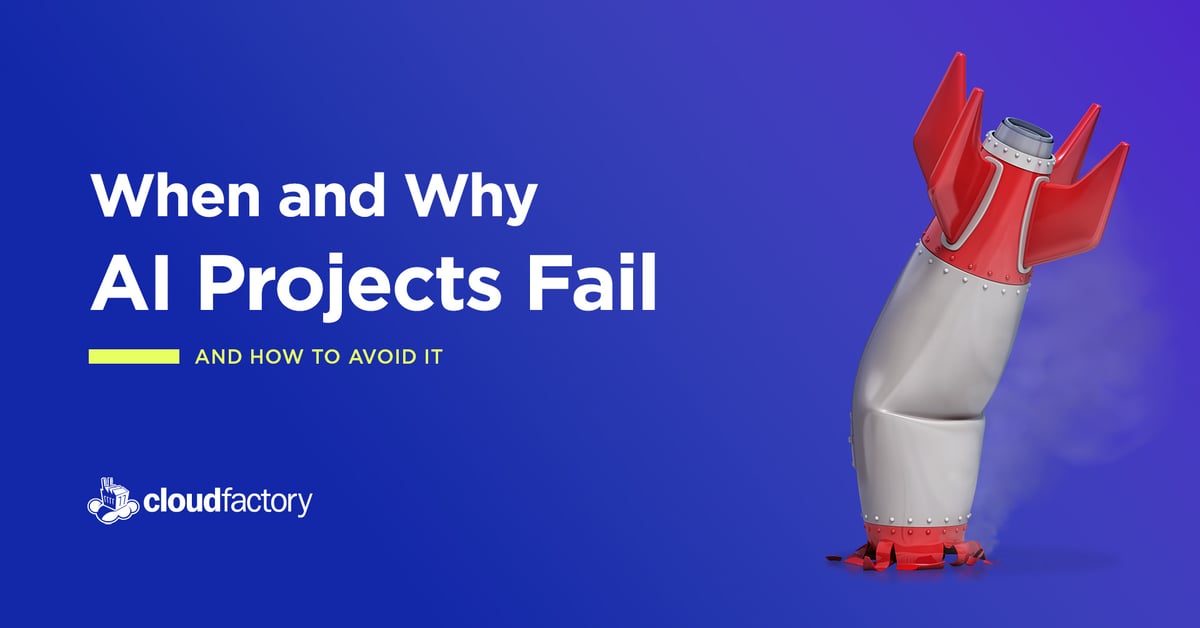
Half of artificial intelligence projects fail for one in four companies, according to International Data Corporation (IDC) research involving 2,473 organizations that are using AI.
At last month’s AI Summit in San Francisco, Melody Ayeli, Toyota’s director of software licensing and complex acquisitions, shared her experiences with AI projects in a discussion session, “Why do AI projects fail?,” led by Will Thompson, managing director of Forbes AI.
Ayeli works in the office of Toyota’s CIO, where she evaluates prospective projects on considerations including software licensing, financial risk, and legal rights. She also has worked on AI projects in a prior role at Deloitte. “I’ve seen AI fail in three phases in the process: early, midway, and later stage,” she said. Here are the problems she said she typically sees during each of these stages.
Early Stage: Managing leadership’s expectations
The primary challenge for AI projects early in the process is managing and communicating expectations to leadership, especially related to the time and cost it will take to develop the technology, Ayeli said. AI projects are not linear, so it’s important to communicate to executives that the process will require time and testing. As development teams train machine learning models and gather new insights, they can iterate and improve the process along the way.
Ayeli shared a story about one AI project that required the creation of a cloud-based data lake before data preparation or modeling could begin. Establishing that data-storage infrastructure took three years.
“When you’re convincing leadership to invest in your AI project and their timeline is this fiscal year or next, it’s hard to get them to support a project that won’t even get off the ground for three years,” she said.
You also need to plan for turnover in leadership, she said, because you may have to sell your project to leadership more than once. “You need a compelling story to share with leaders that shows that without their support of your project, they will miss an opportunity in the market. You want to get everyone on board and communicate clearly the anticipated return on investment,” she said. Keep in mind, when your innovation doesn’t translate into financial benefit, it can be hard to convince leaders to support it.
Midway: Partnership across the organization
When projects fail in the middle of development it is because teams developing AI haven’t partnered with others in the organization who are important stakeholders. “This can cause a delay, when you have to get them up to speed,” Ayeli said.
Tooling and software can become an issue at this stage because deploying newer commercial AI applications in your existing tech stack can cause issues with your original software. “Bringing that large amount of data to integrate with an enterprise application can be a challenge,” she said.
In addition, licensing models for commercial AI systems can be difficult for leaders to understand. The level of investment required in licensing varies but can include fees for licensing, configuration, and users. “It helps to involve other teams who are not part of your project team, because it can be tough for project teams to estimate their costs now and as they move through the development process.”
“The best approach here is to be a fast follower rather than an early adopter when it comes to commercial AI applications,” she said. “It will reduce your risks and potential costs.”
Later Stage: Volume of structured data
Failures in the later stages of AI tend to be data-related, whether it’s quantity or quality - or both. Teams may not have enough labeled data to develop robust machine learning algorithms - or the data may not be high quality enough.
Ayeli shared an example about a team that developed an intelligent customer system to help the company’s field representatives identify add-on projects, or upsell opportunities, that they could offer customers. It took the company 18 months to learn that the field representatives weren’t using the tool, said Ayeli. The system’s limited amount of data wasn’t providing relevant information for the representatives to use.
“That’s a risk. If you don’t have enough data, or if your data is not high quality and it could impact human lives, it may not be worth the risk,” she said.
Ayeli added that having a diverse team across your AI project - from data labelers, designers, and engineers to internal and real-world testers - also is important. “Without that diverse team, you might miss out on discovering the more risky aspects of your project or uncovering what might get in the way of product adoption,” she said.
Key Takeaways: How to Avoid AI Failure at Early, Midway, and Later Stages
You can set up your team for success with these key takeaways from Ayeli’s discussion session:
- Proactively manage and communicate expectations to leadership, especially about the time and resources your project will require. Be sure to consider costs related to technology, data, people, and process. Communicate early and often to ensure everyone understands your project’s progress, challenges, and new opportunities.
- Partner closely with stakeholders in departments across your organization. Be sure to consider software licensing, tooling and its integration with your existing tech stack, potential data migration issues, data feature selection, and cost estimates. Stay close to the teams that work directly with the people who will use the technology, as they will strongly influence the success of your project.
- Don’t cut corners on quality data. Your machine learning model will only be as good as the data that trains it, so select partners carefully and be sure they value and respect data the way your team does. Many of the factors that influence quality are related to your data labeling workforce. Be sure the people who work with your data have labeling expertise and can deliver the high quality you will need to train, validate, and tune your models.
For a decade, CloudFactory’s managed teams have prepared and labeled data for machine learning. In that time, we’ve worked on hundreds of use cases across multiple markets. To learn more about setting your team up for success in developing AI, check out The One Thing You Need Before Starting Any AI Project.