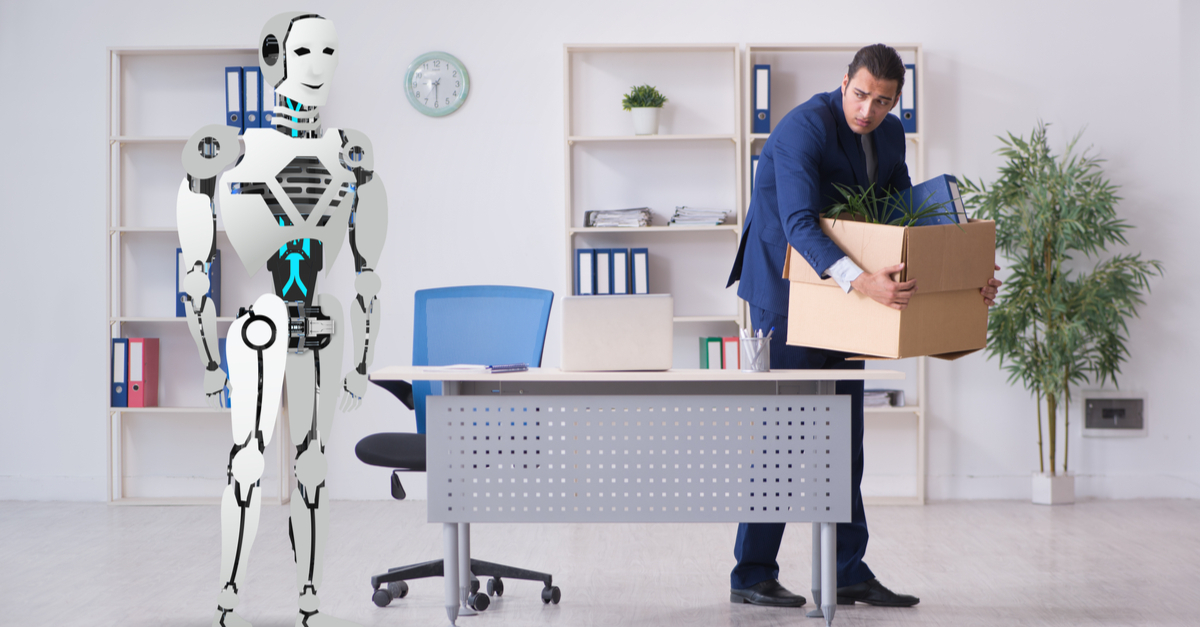
Data annotation might seem like a purely technical challenge. Machine learning is widely seen as a means to replace human effort, and even as a threat to people’s jobs. But these views miss the fundamental point that people and machines perform best when each works together to augment the other’s capabilities.
When most people think of machine learning, they place the emphasis on the machine. However, for the machine to learn, people have to train machine learning models and maintain them in production thereafter. Every successful AI project includes humans in the loop who do everything from training and testing the algorithms to labeling data, conducting quality control, and monitoring automation.
The current and future state of human-in-the-loop AI
AI models are prediction and decision-making engines. Most everyday models have fairly simple tasks to do, such as recommending which movies people might like to watch, based on their viewing history. Others have far greater responsibilities, like medical AI predicting a patient outcome based on healthcare data or an autonomous vehicle making a quick decision to apply the brakes to avoid hitting a person in the road.
Applications like recommendation engines only need to be accurate enough to be somewhat useful. After all, no one is going to lose their life if Netflix recommends the wrong movie or Siri misunderstands a word. It’s a completely different matter for computer vision applications that are trained to assist in critical business functions and areas like healthcare or self-driving cars. For these applications, data quality and accuracy are critical. There’s no room for near-perfect.
This is where humans in the loop (HITL) can assist. HITL applies people in the AI model development process to apply human expertise and judgment during the model development process and across the AI lifecycle. High-performing AI systems rely on humans in the loop.
So, will AI replace humans in the loop? Not anytime soon. Here’s why.
Human judgment and expertise are critical for AI development
Computers have always existed to solve mathematical problems. AI is the next step forward in the development of those solutions. Its goal is ultimately to use data to solve those problems faster and make decisions and take actions based on those outputs.
To be effective, an AI model needs to translate abstract ideas that only people can understand into training documentation that a machine can understand. In other words, it depends on human experience and judgment in the form of intricately prepared data sets and advanced mathematical models. That’s something that can only be achieved with humans in the loop.
Quality control requires human intervention
People play a key role in interweaving quality control throughout the AI development lifecycle. Human judgment and subject matter expertise are critical at every stage of the process. In the early development phases, people need to collect the right data and label nuanced data sets that cannot be understood by a machine.
Quality control continues throughout the production stage to ensure the model can make accurate decisions in changing circumstances. For example, an AI model trained to detect and recognize retail goods will need to be constantly refreshed, given that product packaging changes all the time.
Real-world scenarios are incredibly complex, and translating them into mathematical problems for computers to solve requires constant input from humans. A computer vision model might be able to tell the difference between a hot dog and a sandwich, but not necessarily between a real hot dog and a plastic model of one.
A neural network is only as effective as the training data used to develop it. Machines cannot make sense of nuanced data sets without proper training. In more serious use cases like AI in healthcare, these can lead to severe consequences, such as a misdiagnosis.
Technology assists human annotation workforces
The data annotation tool market is just beginning to grow, and quickly. Auto-labeling can be applied to speed labeling and improve accuracy. However, assisted and automated annotation requires quality control. For example, if an ML model encounters input it cannot identify or reports a confidence level below a certain threshold, people step in to make corrections. This is typically done through a programmatic interface, where the annotation software flags exceptions for review.
Real-time communication should also be embedded to add context throughout the process. For example, in quality control, a data analyst might open a data bug and associate it with a specific pixel in an image before assigning it to an annotator for correction.
Combined with a managed workforce, assistive solutions like built-in communication tools and automated labeling can help scale humans-in-the-loop and drive better results. Data annotation tools will no doubt continue to evolve to match these requirements.
Learn about the future of human-powered annotation in this on-demand webinar.