Overview
Hummingbird Technologies, a precision agriculture platform company, turned to CloudFactory to scale model development for new crops and geographies. Our team looks at drone and satellite images and annotates individual plants of varying sizes and health levels.
Services Used
- Computer Vision Managed Workforce
Read the client story that follows, or download a PDF version you can reference later.
Industry
Agriculture
Headquarters
Company Size
11-50
6x
Growth in Data Labeling Capacity
7
Crop Products Supported
2+
Year Partnership
Editor’s Note: We talked to Hummingbird Technologies CMO, Alexander Jevons, and Senior Data Scientist, Francois Lemarchand for this Q&A case study. Answers have been combined and edited for clarity.
Tell us a little bit about Hummingbird Technologies.
We provide crop analytics through machine learning algorithms applied to remote sensing captured imagery, captured by both drones and satellites. The idea is to help our customers increase their yields, use the optimal amount of inputs, and farm more sustainably. We have created 70 different ML based products with 90%+ accuracy and our customers typically see an increase in their agrochemical efficiency by 20-30% on average.
How did Hummingbird get started?
Our founder, Will Wells, realized while he was studying for his MBA at INSEAD that the standard practice of over-spraying chemicals on crops just wasn’t sustainable from an ecological, environmental or political standpoint. He figured out that remote sensing from drones, planes, satellites and robots, combined with artificial intelligence, could reduce the use of chemicals, but also help increase crop yield, save farmers money and just, really, help the agriculture industry in general.
The goal was to deliver products that farmers would embrace, products that solve real life problems. We want to help farmers save the environment without any detrimental impact on yields and their livelihood. We started in the U.K. and then began offering services in Brazil, Australia, Ukraine and Russia. This year we’ve expanded to Canada and we’ve just signed our first contract in Malawi.
Can you explain a little bit more about what information you provide farmers?
Whether it’s deciding how much nitrogen to put down, when or how much herbicide or fungicide to use, or where and how quickly a crop is growing, we have developed models that provide farmers with detailed information. All of it is crop specific - since no two crops are alike - and all of it is agronomy and AI-led. Farmers can even send the application information to their machinery to automatically adjust inputs.
Our products help farmers reduce inputs, increase crop yields, or, on occasion, both. One of our client farmers has told us he is now, “Farming to potential, not hope.’’
So you mentioned that all of your models are crop-specific, what goes into creating a model for a new crop or product?
When we are considering adding a new crop to our product line, or adding new products for crops we already cover, we first meet with our in-house agronomists to have a better understanding of the different variables (seasonality, growth, disease, etc.). Weighing this subject matter expertise along with drone imagery of the new crop allows us to evaluate the feasibility of a new product. We are sometimes able to use similar, existing AI models (for example a model that counts lettuce heads being used to count similar crops) as a starting point. Nonetheless, it makes sense to have a dedicated model for every task and crop to obtain optimal results.
Once we determine if we want to move forward, we then have to decide whether this will require real-world measurements from our agronomists in the fields, or whether we can utilize image annotations. That’s where the CloudFactory team comes in. The great thing about using a dedicated team of annotators paired with deep learning techniques is that the time required to build models is reduced as we increase our library of knowledge and models. In addition, we are now pre-annotating data using similar, existing models before the team corrects the AI-generated annotations, significantly increasing productivity.
With the help of CloudFactory, we’re being a lot more ambitious with our data sets. We have the freedom now to spend 400 hours annotating a large data set, because it isn’t taking up the time of internal resources. Before partnering with CloudFactory, there was no way one of our four data scientists was going to spend six months labeling data. It was just not possible at all.
Francois Lemarchand
Senior Data Scientist
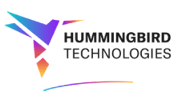
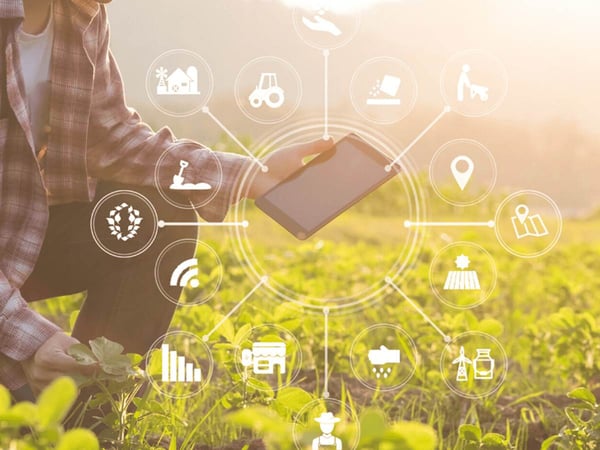
How do you update models to account for fluctuations in climate or other irregularities?
I can give an example from our product that counts and sizes lettuce heads in drone imagery. We trained a model as a proof of concept for one of our clients. We ran some tests and the model was very successful during the first season. Then we had a second season where, because it was raining so much, farmers had to use a thin plastic sheet called a fleece to protect the plants. We had to retrain the model to teach it to recognize the plants through the fleece. It worked and the entire process to upgrade the model took only a couple of days.
To make our models more robust, we use a technique, called data augmentation, which consists of modifying our training data in such a way that it will force the model to focus and learn new characteristics of the data. For instance, we can apply a blur effect or convert to grayscale a small percentage of our images when feeding it to the model. This will allow the model to recognize plants even if we encounter minor issues with the drone’s camera or if an unusual crop variety is growing in the field.
How is CloudFactory different from your previous annotation processes?
One of our key challenges was tagging all the data we captured and making sense of all it so we could build our models. As mentioned previously, it is highly domain specific knowledge. We had been doing the work in-house but it is very, very time-consuming. The question was how could we teach annotators how to do this without them being agronomists, experts who study soil and crop management. What we discovered is that you need to iterate and have a continuous exchange of communication, which is something we can do with the CloudFactory team.
With the help of CloudFactory, we’re being a lot more ambitious with our data sets. We have the freedom now to spend 400 hours annotating a large data set, because it isn’t taking up the time of internal resources. Before partnering with CloudFactory, there was no way one of our four data scientists was going to spend six months labeling data. It was just not possible at all. We now have 10 members in the R&D team and our ambition is to have every single team member linked directly to the CloudFactory team to make the process even smoother. As we are an ever growing startup, it also helps us massively to be able to ramp up gradually the number of hours as new projects are coming in.
Can you walk us through a specific project you’ve done with us?
The largest project we have completed with CloudFactory to date is the lettuce plant sizing project mentioned earlier. This product helps farm managers identify areas where the crop performs the best so that they can optimize their harvest and limit waste when spraying chemicals. The CloudFactory team looked at high-resolution drone images and labeled all of the lettuce heads of different sizes so that our model could, basically, tirelessly repeat the task forever. It took a significant amount of time to kick off this project initially as the team had to familiarize with drone imagery of crops, but that was something we expected since it’s not something you see every day!
It can be quite difficult to stay motivated as an annotator when you are looking at imagery of a field with over tens of thousands of plants and we try to progressively increase the labeling tasks’s difficulties as the project happens so the Cloudfactory team can start to make their own subjective decisions. It was great to see the team asking, for example, questions about whether some plants may be healthy or not - and the plants were actually dead. These questions and the feedback loop we have with Cloudfactory ensures that the same errors do not show up over and over again in the dataset, since we are managing and giving guidance on edge cases in real time. As soon as the dataset was in a well advanced stage, I started working on the data to build a prototype of the model and was able to share with the team what they had contributed to create and how this will impact the world.
How critical is accurate labeling to AI and Machine Learning?
In our specific case, our AI models are providing information directly to decision-makers in agriculture. Therefore, it is important that the information is reliable since you don’t get a second chance. If a farmer trusts you, they need to be sure that crops will still perform the same after they take the suggested action from our products, as a loss of yield could result in grave consequences for their financial health. This could also lead to a much more dramatic impact on a regional scale. For this reason, the farming community is understandably cautious when investigating new technologies.
The problem with labeling is that agronomists are far too in demand in the fields to be able to assist us. The second best solution is that we consult them and draw a list of requirements together before we teach annotators. The annotators are then advised to flag every doubt during the labeling process so a data scientist or agronomist can help make a decision. While we cannot totally exclude human error during a labeling process, AI techniques allow us to generalize main rules from the data, meaning all one-off mistakes will be ignored. Regardless of this tolerance for error, it is critical to have the best annotations possible so that repeated errors do not occur and impact the model.
How do you think AI is going to evolve to help agriculture?
I think that the farming community will soon become more comfortable and knowledgeable about the potential for AI and AI-based, remote sensing products. The sort of Holy Grail that we always get asked about is pre-symptomatic disease detection. That is incredibly hard, if not impossible, to do at the moment. But we definitely see this happening with more sophisticated technology and greater improvements and accuracy. You can go from being right 85-90% of the time, but our customers will want 98%, 99% of the time. And that will definitely, definitely happen.
We're already setting the state-of-the-art in several products and we are very confident that within the coming years, the products that Hummingbird and CloudFactory develop will be seen as the industry standard. And then, in my opinion, if you're not using them you'll be seen as behind the times. It’s going to come to the point where, if you're using the industry practices that remain as standard in early 2020, then by 2023 you won’t survive.
You mentioned pre-symptomatic disease detection as something that your clients want. When you think about future applications of AI, what are some of the things you are most excited about?
While Hummingbird is developing cutting-edge products for both drone and satellite imagery, many of our competitors have given up on drones. That is why some of our drone-based products are unique and we are looking forward to a future where drones become even more popularised and automated. Drones can provide aerial images where a single pixel represents a millimetre in the real world, which is an impossible thing for satellites. You could then have farmers launching their drone in the afternoon and then use the data produced by Hummingbird to spray with a variable rate the next morning.
In addition, it almost seems like science fiction at this point, but working with LIDAR data from a drone would be really exciting. LIDARs are usually used by self-driving cars to estimate distances from an object while we mostly use optical imagery. At this point, it is extremely expensive and not commercially scalable but you could imagine mapping a field, for example, and getting an accurate height of the crop across your wheat field. We also tend to hear a lot about the next camera upgrade of the next hot-and-coming smartphone, we know exactly the same excitement in sensors for drones and satellites. The sensors improve in ways that would not be considered traditional. We own one of the only hyperspectral cameras for drones in the UK and this camera can record non-visible information (e.g. infrared) at a very high resolution. This already opens doors for new research projects investigating early-stage disease detection and new ways to measure crop health.
Recommended Reading
We have 10+ years of experience helping our clients focus on what matters most. See what we can do to help your business.
Precision Agriculture
Technology holds great promise for solving the many challenges and inefficiencies in the production and distribution of food.
Geckomatics
Geckomatics turned to CloudFactory to help scale their cost-effective solution to more customers around the world.
Robotic Automation
The combination of AI and robotics can improve outcomes in healthcare, agriculture, manufacturing, and more.
Contact Sales
Fill out this form to speak to our team about how CloudFactory can help you reach your goals.