The only shortcut to data labeling success is to do it right the first time.
Don't be fooled into thinking that any old data labeling workforce will give you the quality data your AI project needs to optimize your ROI and outperform your competition.
You need the right workforce from day one and need to know what specific traits to look for.
With over a decade of experience providing managed data labeling teams for startup, growth, and enterprise companies, we’ve learned four workforce traits that universally affect data labeling quality for ML projects.
We're talking about expertise, agility, relationships, and communication—key elements we cover in our comprehensive data annotation guide, which helps you choose the right provider, streamline processes, make the most of technology, conduct quality assurance, and avoid common pitfalls.
1. Expertise
In data labeling, expertise is essential for your workforce to create high-quality, structured datasets for ML. This is what we call the art and science of digital work. It's knowing how to integrate people, processes, and technology to achieve your desired business outcomes.
There will be trade-offs, and your data labeling service should be able to help you think through them strategically. For example, you’ll want to choose the right QA model that balances quality and cost with your business objectives.
We’ve learned workers label data with far higher quality when they have the context or know about the setting or relevance of the data they're labeling.
To tag the word “bass” accurately, they will need to know if the text relates to fish or music. They might need to understand how words may be substituted for others, such as “Kleenex®” for “tissue”.
For the highest quality data, labelers should know key details about the industry you serve and how their work relates to the problem you are solving.
If you want to create the best data annotation guidelines for your data labelers that drive accurate ML models, then don't miss this article.
It’s even better when a member of your labeling team has domain expertise or a deep understanding of the industry your data serves so they can manage the team and train new members on rules related to context, the business, and edge cases.
For example, the vocabulary, format, and style of text related to retail can vary significantly from that of the geospatial industry.
2. Agility
ML is an iterative process. Data annotation evolves as you test and validate your models and learn from their outcomes, so you’ll need to prepare new datasets and enrich existing datasets to improve your algorithm’s results.
Your data labeling team should be flexible enough to incorporate changes that adjust to your end users’ needs, changes in your product, or the addition of new products.
An agile data labeling team can react to changes in data volume, task complexity, and task duration. The more adaptive to change your labeling team is, the more ML projects you can work through.
As you develop algorithms and train your models, data labelers can provide valuable insights about data features - the properties, characteristics, or classifications that will be analyzed for patterns that help predict the target or answer you want your model to predict.
3. Relationship Building
In ML, your workflow changes constantly. You need data labelers who can respond quickly and make changes in your workflow based on what you’re learning in the model testing and validation phase.
To succeed in this agile environment, you need a data labeling company that can adapt to the processes for your unique use case while also having the experience and expertise to know what processes are needed in the first place.
It’s even better if you have a direct connection to a leader on your data labeling team so you can iterate data features, attributes, and workflow based on what you’re learning in the testing and validation phases of ML.
4. Communication
Direct communication between your project team and data labeling team is essential. A closed feedback loop is an excellent way to establish reliable collaboration.
Labelers should be able to share their insights and feedback on common edge cases as they label the data so you can use their insights to improve your approach. When multiple teams are involved, each should have a dedicated team lead or project manager to coordinate with the data annotators.
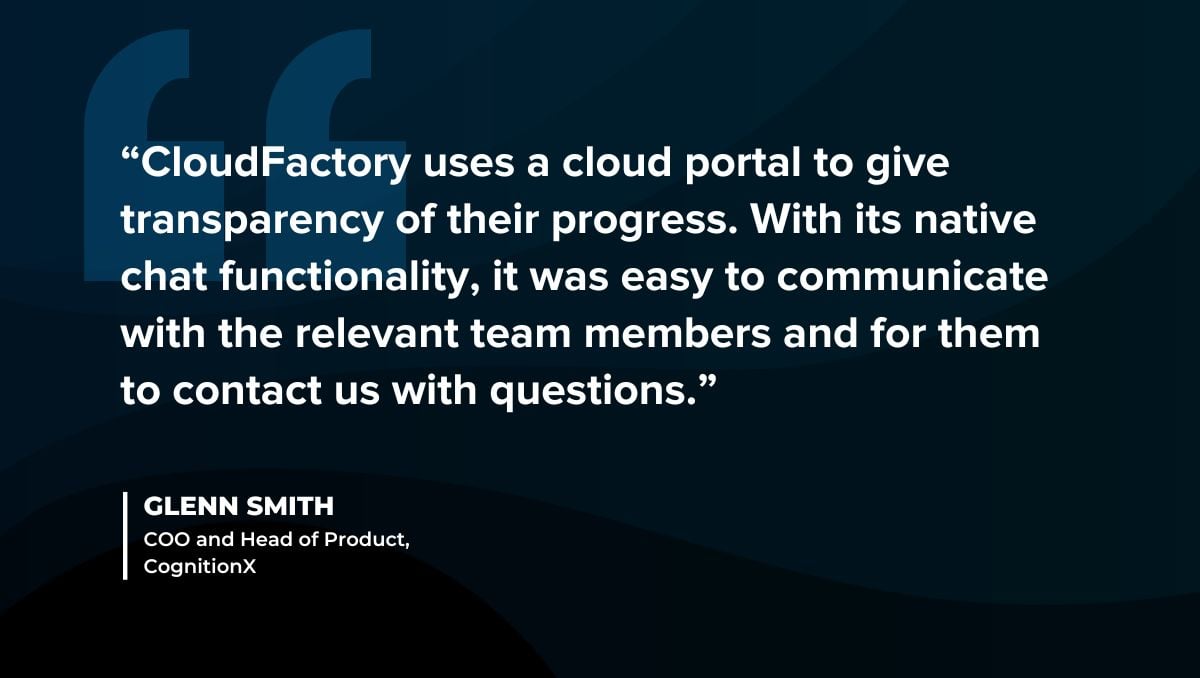
Your partner for high-quality data annotation
CloudFactory has helped its clients create high-quality datasets that train, sustain, and augment AI models for over a decade with a powerful mix of human experts and leading workforce management technology. We provide experts who are dynamically matched to your project needs that support and scale with your most time-sensitive, vital tasks.
If you're new to data labeling and want the top tips on managing vendor relationships and setting them up for long-term success, check out our white paper, Your Guide to AI and Automation Success Through Outsourcing.