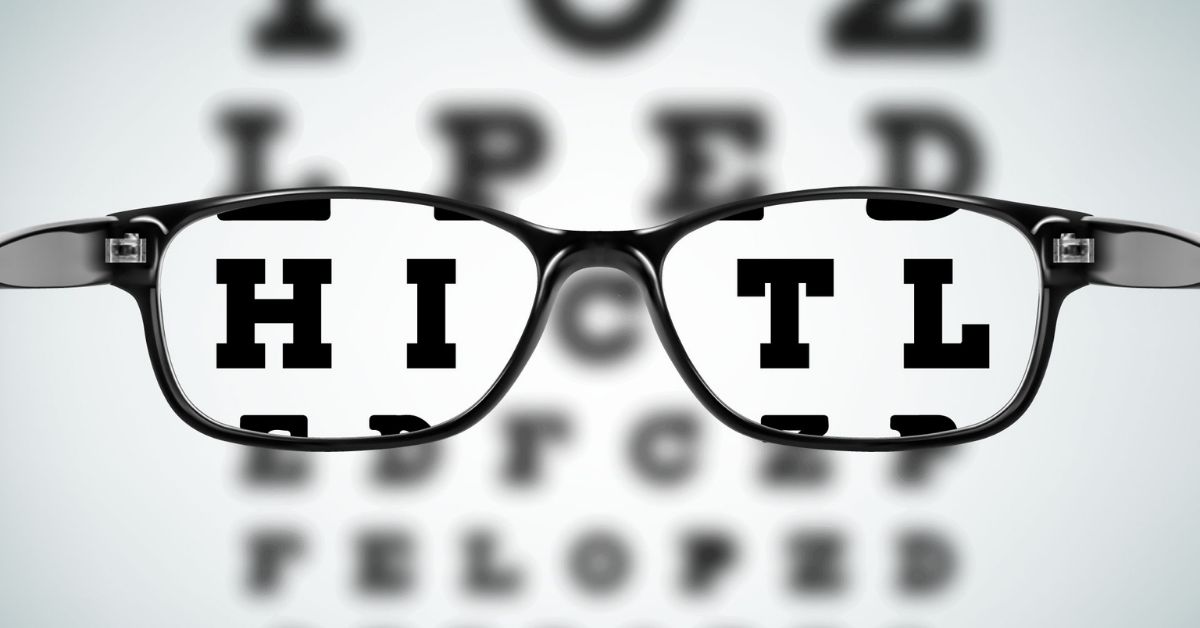
In the late 1920s, a self-taught Austrian engineer named Gustav Tauschek developed a mechanical device with a photoelectric cell that used template matching to transcribe handwritten text into printed characters. His Reading Machine was a result of decades of development beginning with the transliteration of written telegrams into Morse code beginning in the 1870s. The invention was early-stage optical character recognition (OCR).
After nearly a century of advancement, OCR has come a long way, developing in line with the hard disk drive, automatic test scoring equipment, and office computers. Today, OCR software is largely an evolution of the system developed by American inventor Ray Kurzweil in 1976. His work was born out of an interest in applying pattern recognition to expand the printed materials available to non-sighted readers. Kurzweil invented his reading machine by developing a flatbed scanner and full text-to-speech program. It was demonstrated for the world in 1976, when it read Walter Cronkite’s sign-off at the end of a CBS news broadcast.
OCR Accelerates Data Entry for Digital Transformation
OCR software is now widely available to businesses and consumers. It’s a standard software bundle included with some all-in-one office printers. OCR is built into popular note-taking apps, such as Evernote, and scanning apps on your smartphone. The U.S. postal service has been using it to sort mail since 1965, and OCR enjoys an established reputation for easing data entry and transcription in finance, healthcare, and other industries.
Today’s OCR solutions can transcribe printed and handwritten characters in hundreds of different languages. It has become the standard for parsing data from scanned documents into editable, searchable text. OCR’s accuracy has improved enormously over time, lending to greater productivity and substantial cost reductions for organizations that use it in place of manual data entry.
OCR has eased the digital transformation for healthcare, finance, and other industries as workers transform written documents into searchable digital data. It continues to play an important role in the gathering and digitization of data, as many organizations still rely on handwritten records and documents that are generated in real time, such as transaction receipts.
OCR Error Rates Are Up to 20%
For all of the benefits of OCR, it comes with a caveat. As with most automation, 100% accuracy is not possible. Generally, OCR software error rates hover from 2% to 20%, with older documents being the most challenging to transcribe accurately.
It’s important to keep in mind that an OCR error rate of 3% might be acceptable for a small dataset. But that error rate across a corpus, or large collection of written texts, may not be acceptable. Further, one study assessing the impact of OCR errors in information retrieval found that statistically significant quality degradation begins at a word-error rate of 5%. These are all aspects to consider when using OCR software.
Here are some of the factors that contribute to OCR errors:
- Handwritten text: The highly variable nature of handwritten text, even including forms filled in using block capitals, is harder for a machine to interpret than computer-generated text.
- Special symbols: Transcription of symbols, such as those used in mathematical equations, can result in high error rates.
- Characters: Languages use different characters. Some use diacritics, or signs above and below letters, to indicate differences in pronunciation.
- Unclear text: Text that is difficult to read, such as blurred printing or a coffee stain that covers numbers on a receipt, can affect accurate OCR transcription.
- Complex fonts: Most OCR software can recognize any standard typeface but may encounter exceptions with complex fonts that require special interpretation, such as cursive characters.
OCR Requires People to Monitor for Quality
While OCR can be designed to address and improve quality relative to specific types of errors, a percentage of data it processes will require manual inspection and moderation to ensure high quality.
Whenever an automated solution like OCR reports a confidence rate below a certain predefined threshold, it will flag the outcome as an exception that a person will manually review.
Enter the other half of this dynamic data-entry duo: people. The people who use and monitor OCR solutions play an important role in ensuring quality data outputs.
These humans in the loop (HITL) bring two benefits to the process:
- They ensure quality control by processing exceptions, and
- Their actions can be looped back into the system to inform improvements.
The HITL approach is vital for high-volume data-entry and OCR workloads that require accuracy. Even a small error rate for a financial institution can result in millions of dollars in lost revenue or noncompliance with regulatory requirements.
Cash-back app Ibotta uses OCR to match items on a receipt with items a shopper selected in the app. The process happens in near real time and needs to be fast. And to maximize value for retail consumers and Ibotta’s partner brands who offer rebates on the app, the process must be accurate. Its proprietary OCR flags some receipts for a person to verify. For what amounts to thousands of receipts a day, Ibotta relies on people to moderate and resolve the toughest cases.
Quality Control Requires Quality People
Indeed, people are the driving force behind intelligent automation like OCR. And to optimize your return on investment from automation, even in fairly simple use cases, you will need a layered quality control process that includes people who can perform manual data entry with high quality and consistency across your data stores.
If you are doing this work in-house, you may be surprised at the many benefits of outsourcing your operations to a managed workforce that has experience with data processing across use cases and industries. A managed workforce can become an extension of your team, ensuring close communication and visibility to the work that is being done. It leverages the best of the dynamic data-entry duo.
To learn more about outsourcing exception handling, data entry, and transcription at scale, check out our helpful guide.
Outsourcing Data Transcription Data Labeling Automation & Back Office Support