As your AI and machine learning (ML) projects grow, so does the volume and complexity of your data. You'll need the flexibility to adjust your workforce capacity without sacrificing data quality, especially since ML demands constant iteration and refinement for optimal model performance.
Tasks like video annotation highlight this key challenge—annotating a single hour of footage can take up to 800 human hours!
Your company may be experiencing any of these scenarios:
-
Your high-value resources are bogged down:
Data scientists and ML engineers are better suited for strategic analysis and extracting business insights from data than repetitive labeling, which is a less effective use of their expertise and time. -
Your data volume outpaces capacity:
If data volumes grow over weeks or months, your in-house teams might need help to keep up, leading to delays and potential bottlenecks in your ML and data analysis projects.
Therefore, we recommend following these five steps to keep up the pace and successfully scale data labeling:
- Design for workforce capacity
- Look for elasticity to scale labeling up or down
- Choose smart tooling
- Measure worker productivity
- Streamline communication between your project and data labeling teams
Let’s look at each individually as you examine the capacity of your current labeling operations to handle project expansion and the rate of business growth effectively.
1. Design for workforce capacity
When it comes to data labeling, there are a few options available, including using a data labeling service or crowdsourcing. However, it's important to note that using anonymous workers through crowdsourcing can come with risks, such as lower-quality data. That's why working with a managed team of labelers who deeply understand your business rules, context, and edge cases can be a better option.
Having a consistent team of labelers can improve data quality as they become more familiar with your needs and can even train new team members. This is especially useful for ML projects, where high-quality data and the ability to iterate quickly are essential.
2. Look for elasticity to scale labeling up or down
Labeling must be based on the volume of incoming data generated by your products' end users. If your business experiences seasonal spikes in purchase volume, for example, during certain weeks of the year, you may need additional labeling support, especially before the gift-giving holidays. Similarly, you may have to label a significant amount of data during the growing season or product launch to ensure your models are up-to-date.
Ensure your workforce can adjust and scale based on your specific needs to handle such spikes.
CloudFactory has extensive experience helping clients manage surges in data labeling volume with great success.
For instance, we recently assisted a client with a product launch that required 1,200 hours of data labeling within a tight five-week deadline. We completed this demanding task on time and within budget, enabling the client to focus on other crucial aspects of their product launch.
Another client needed to develop innovative mobile solutions quickly to test and develop new retail AI use cases. Launching a minimum viable product (MVP) was essential, yet the costs and resources required for internal labeling software and managing a team of labelers posed a significant challenge.
To overcome this hurdle, our Accelerated Annotation platform proved to be the game-changer. It helped the client launch their MVP within two months while maintaining their competitive edge.
3. Choose the right tooling
Selecting the right data annotation tool can significantly impact your ability to scale data labeling, including the possibility of utilizing automated data labeling approaches. As data labeling tasks evolve over time, it's essential to have a tool that can grow with your business.
Whether you're starting from scratch or operating at scale, the right tool will allow you to iterate on labels, enhance AI model performance, and move into production. While in-house tools offer the most control, commercially available tools include best practices from thousands of use cases, making them highly flexible and customizable.
With a third-party tool, you can focus on growing and scaling your data labeling operations without the overhead of maintaining an in-house tool.
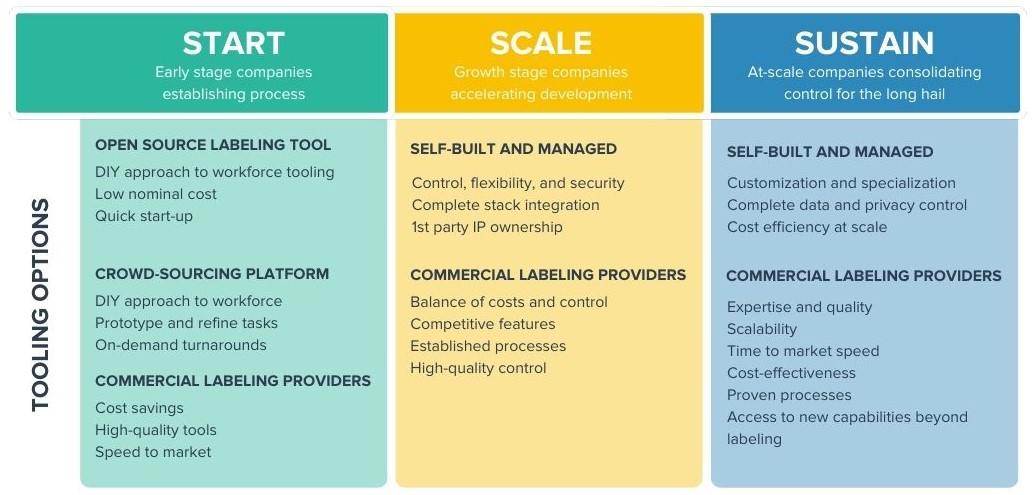
4. Measure workforce productivity
Your data labeling workforce's productivity is influenced by three key aspects: the volume of work completed, the quality of work (accuracy and consistency), and worker engagement. In this case, achieving process excellence involves combining technology, workers, and coaching to shorten labeling time, increase throughput, and minimize downtime.
Our experience has shown that data quality is significantly higher when data annotators are placed in small teams. We have consistently achieved outstanding results by training them on your specific tasks and business rules and providing them with high-quality examples of what good work looks like.
Small-team leaders encourage collaboration, peer learning, support, and community building, which helps to create a positive work environment where workers' skills and strengths are known, valued, and allowed to grow professionally. The approach and a smart tooling environment result in high-quality data labeling. Workers use the closed feedback loop to deliver valuable insights to the machine learning project team, which can improve labeling quality, workflow, and model performance metrics such as mean Average Precision or accuracy score.
A workforce management platform is essential to further enhance worker productivity. The platform should enable task matching based on worker skills and supply-demand management to allow flexible distribution and management of large volumes of concurrent tasks, performance feedback to support continuous improvement of labelers, and a secure workspace to adhere to the strictest security practices.
5. Streamline communication between your project and data labeling teams
CloudFactory implements a closed feedback loop to ensure seamless communication between you and your labeling team. This allows you to modify your labeling workflow, iterate data features, and achieve your desired outcomes faster.
In cases where data labeling directly impacts your product features or customer experience, your data labeling team's response time is even more urgent. Our labelers work across different time zones, ensuring optimal communication with your team regardless of location. This way, you can be sure that your machine learning project's end-users receive the best possible experience.
Critical questions to ask your data labeling service about scale:
- Can you explain how scalable your workforce is? How many data annotators can we access at any given time? Can we easily adjust the volume of data labeling based on our specific needs? And how frequently can we make those changes?
- How do you measure your workers' productivity? How long does it take for your team of data labelers to reach maximum efficiency? Does the throughput of tasks get affected as your data labeling team scales? And how do you ensure that the increased throughput doesn't impact the quality of the labeled data?
- How do you handle the various iterations and changes in our data labeling features and operations as we scale? Do you have a process in place to manage this effectively?
- What is the level of client support we can expect once we start working with your team? How often will we have meetings to discuss progress and any concerns? And how much time should our team plan to spend managing the project on a regular basis?
CloudFactory is your scalable data labeling partner
Scaling your ML data labeling operations is essential to keep up with the ever-increasing demands of your AI projects.
But how do you ensure accuracy, speed, and quality as your data volumes grow?
CloudFactory's Accelerated Annotation offers a powerful solution, combining the precision of human expertise with the speed of cutting-edge AI to deliver high-quality labeled data at an unprecedented scale.
Speed up labeling:
Achieve labeling speeds up to 30x faster than manual approaches.Versatile AI assistance:
Tackle diverse image and video annotation tasks (image tags, bounding boxes, segmentation, keypoints, and more).Gain critical insights:
Proactive feedback identifies AI model weaknesses and refines labeling strategies.Tap into proven expertise:
7000+ expert labelers assigned to project-specific needs and 700+ clients with a 70 NPS score for excellent service (10 points above the industry average!).
As you research practical ways to streamline your entire labeling process and achieve AI project success, check out our resource, Mastering data labeling for ML in 2024: A comprehensive guide. It will empower you to utilize the latest tools and techniques and unlock the full potential of your data.